Where: Katoomba to Mittagong (via Hill Top for reasons below)
When: 2020/12/27 18:00 to 2020/12/30 morning
Distance: 131km, with maybe 30 km of buckwhacking (details below).
Conditions: Day 2 was pretty weird, as it started quite hot, probably around 27C, and then it just opened up and poured. Forecasted to unload 20mm, and I think that felt about right.
Useful Pre-Trip Information or Overview: I found a few GPX tracks and added a several backup tracks in Gaia GPS, but opted to follow the boring “quicker” route. I think the GPX I used was not one that had been recorded, but just one that had been created. This was one of the planning mishaps for my adventure: I overestimated possible pace. The GPX had 5km/hr average. I read previous trip reviews of people saying they could jog the track. It quickly became apparent there was no way anybody would be able to do parts of the track that quickly, so either I went a different way, the track got more difficult, or I haven’t mastered bushwhacking.
A few other prerequisites: I checked that the parks I needed to cross weren’t closed, checked the Cox’s River water gauge at Kelpie Point (0.29m), as well as potential water sources. The most challenging water situation would be on Scotts Main Range, and sure enough, I had to use the water pits, which were pretty dirty. David Noble’s site quoted the walk in the Nattai Valley as “mostly this is easy walking on the riverflats”… more on that later.
My goal was to do it in about 2 1/2 days, but with a backup plan to stay one more night, and/or bail via Hill Top.
The Report: I will try to be concise, but include information if anybody else wishes to do this hike. Considering I think some of the parks only opened quite recently, it doesn’t look like anybody has done this track in quite some time. The conditions I encountered were vastly different from the trip reports I have read previously.
Day 0 (21km)
At 17:30 I got off the train and took a cab to Narrowneck Carpark & Gate ($20), and started that long boring walk along Narrowneck. Tarros Ladder has some metal holds, so is pretty easy if you have a light pack and have done some rock climbing, but probably need to be careful if you have a heavy pack. Then over Tarros Ladder Medlow Gap Walking Track, which is now pretty overgrown, and I think next time I would just continue taking the road, but maybe that’s because I was night hiking. Finally, Medlow gap down through White Dog Ridge Firetrail to Kelpie Point Trail, then camped just before Cox’s River.
Strava: https://www.strava.com/activities/4536566086
Photos: https://imgur.com/a/Q6Fl4fR

Looking Fresh
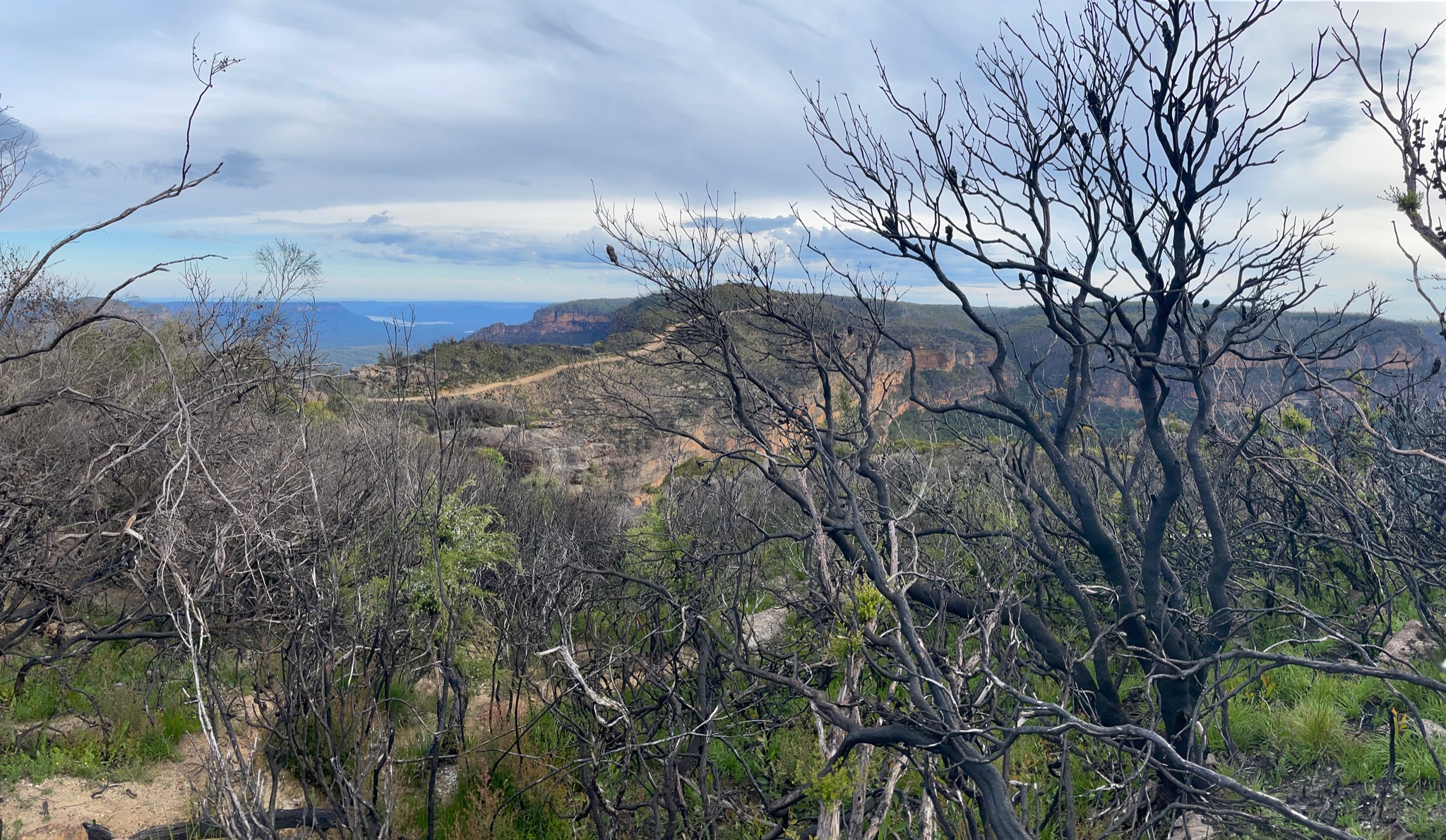
View from Narrowneck
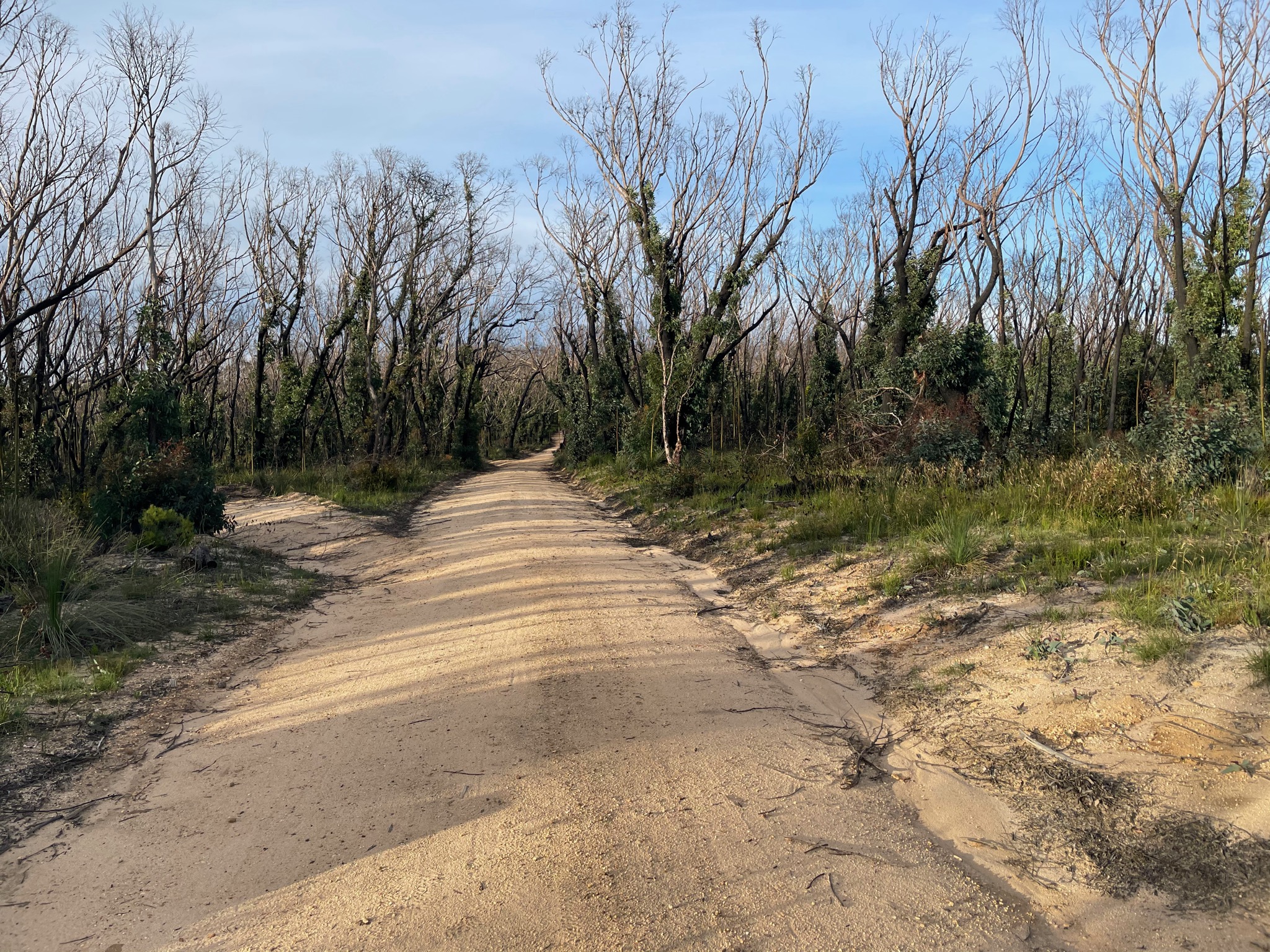
More Narrowneck
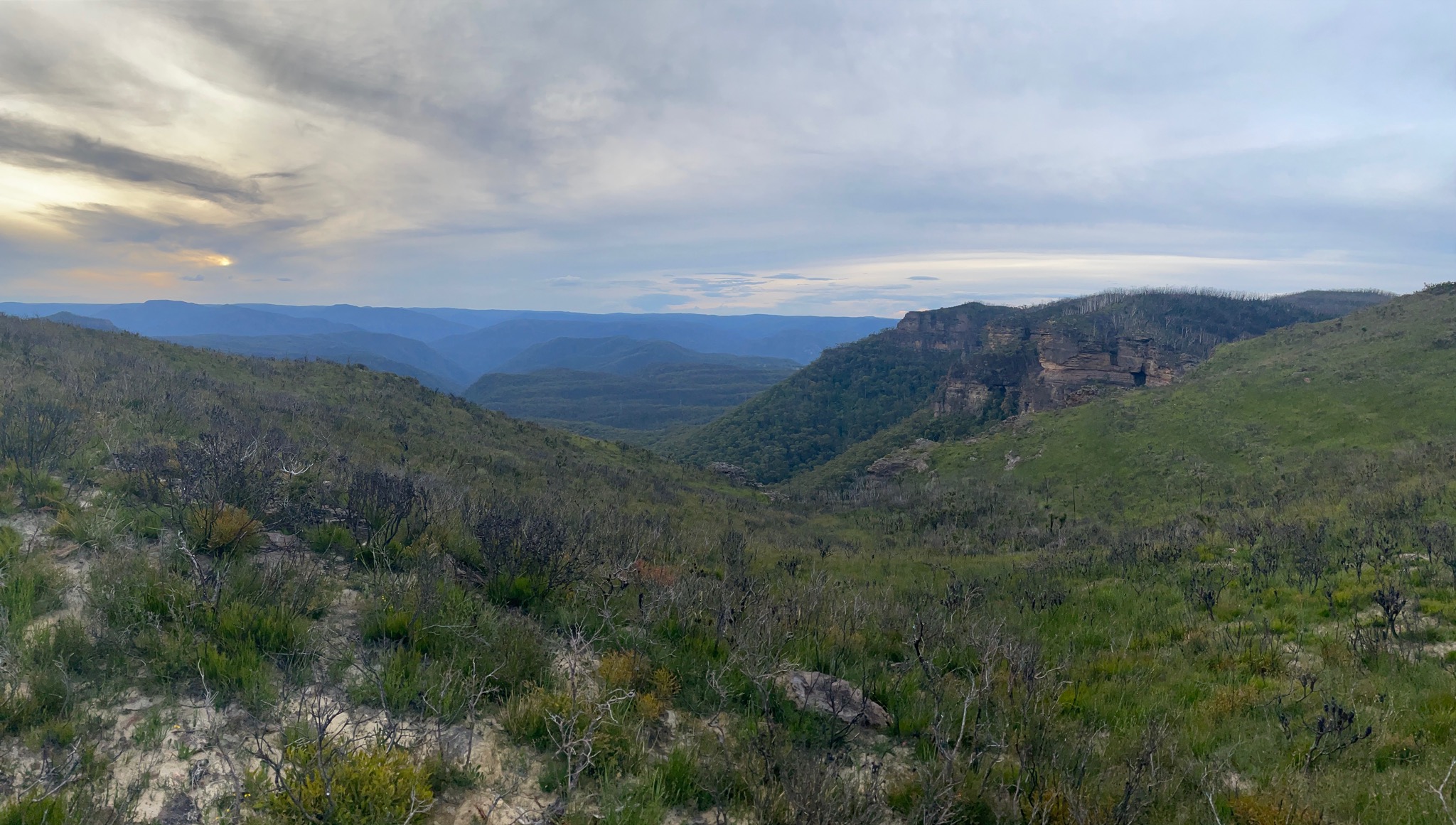
View back towards Katoomba
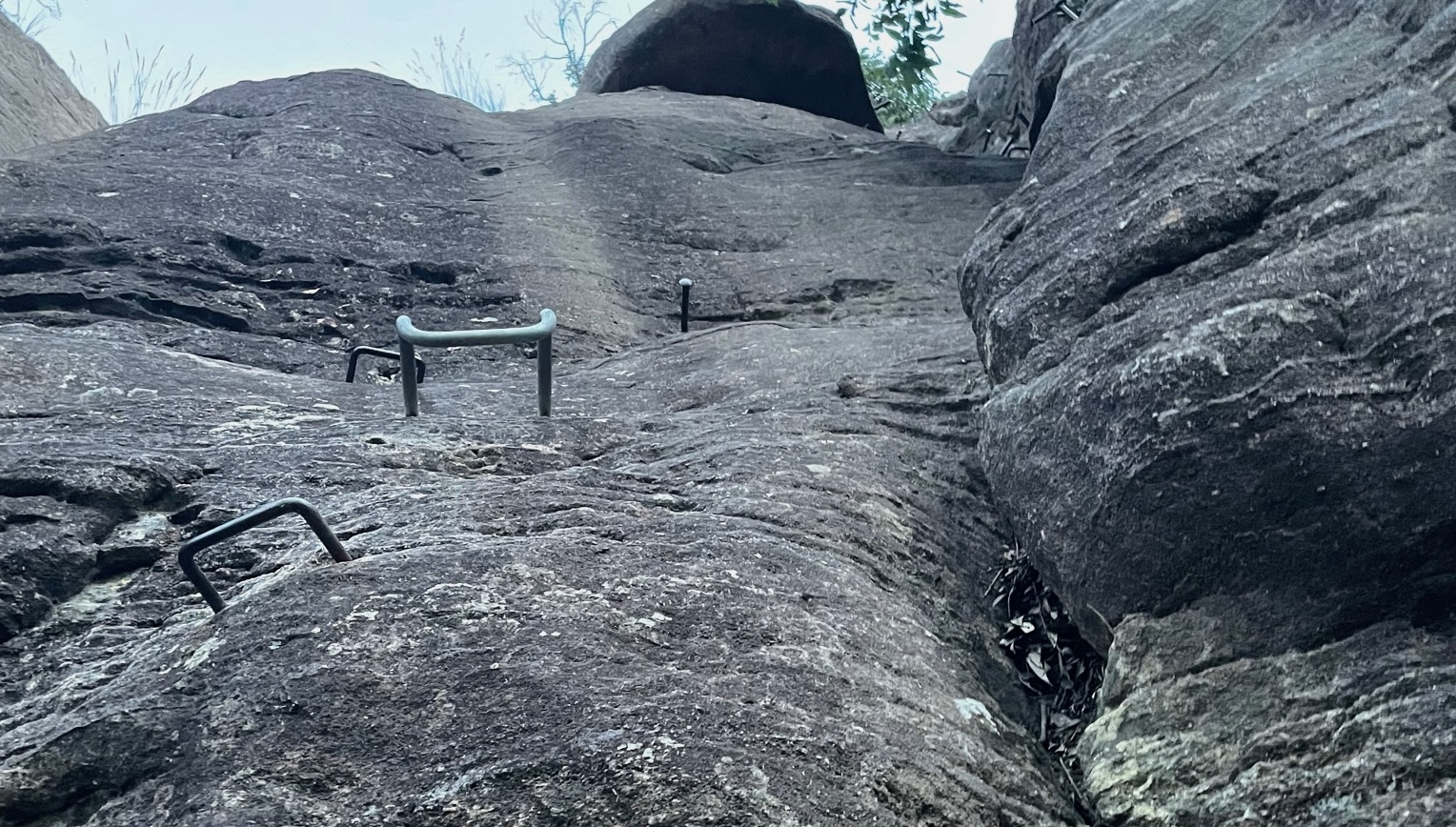
Pretty easy climb
Day 1 (42km)
The day started off on the wrong foot, as I decided to walk the Cox’s River before heading up to Mount Cookem. I contemplate in hindsight if I should have gone back up Kelpie Point Trail and come down and crossed Cox’s River a bit downstream, but the walk along the river only took 40 minutes. The problem is that this type of bushwhacking - waist height to above my head - is pretty draining. Additionally, I’m not certain if I could have crossed downstream after the Cox merged with the Kowmung River, as when I tried, the water was quickly above my waist. Eventually, I first crossed the Cox then crossed the Kowmung, then started up to Mount Cookem. I think I came up the the wrong hill, as I had to do a few pretty gnarly rock climbs. Eventually I popped out and hit Scotts Main Range.
Then a long long fireroad walk. The water wells had water, but they quickly clogged my BeFree. Overall just hot and sort of boring, but the most annoying thing was these big flies that would bite me whenever I stopped. They even managed to bite though my clothing.
I started to just wish for something different, and then a downpour started. Probably 3-4 hours of rain, and everything started flooding. No longer was it a problem finding water, as there were now streams forming in the road. The slightly scary part was that there was lightning hitting pretty close, which I didn’t like.
Eventually I managed to come down through Byrnes Gap, and camped between there and Yerranderie, which was my goal for the day. I probably could have gone further, but the rain was starting to get tiring, and I had a big blister forming (more details in the gear review below).
Remember to look out for dead branches before camping, especially after all the fires.
9hr 19min moving time, but 11hr elapsed time, and at 13:11/km pace (inc the bushwhack and hike up to Cookem).
Strava: https://www.strava.com/activities/4536592392
Photos: https://imgur.com/a/rvIcBkX
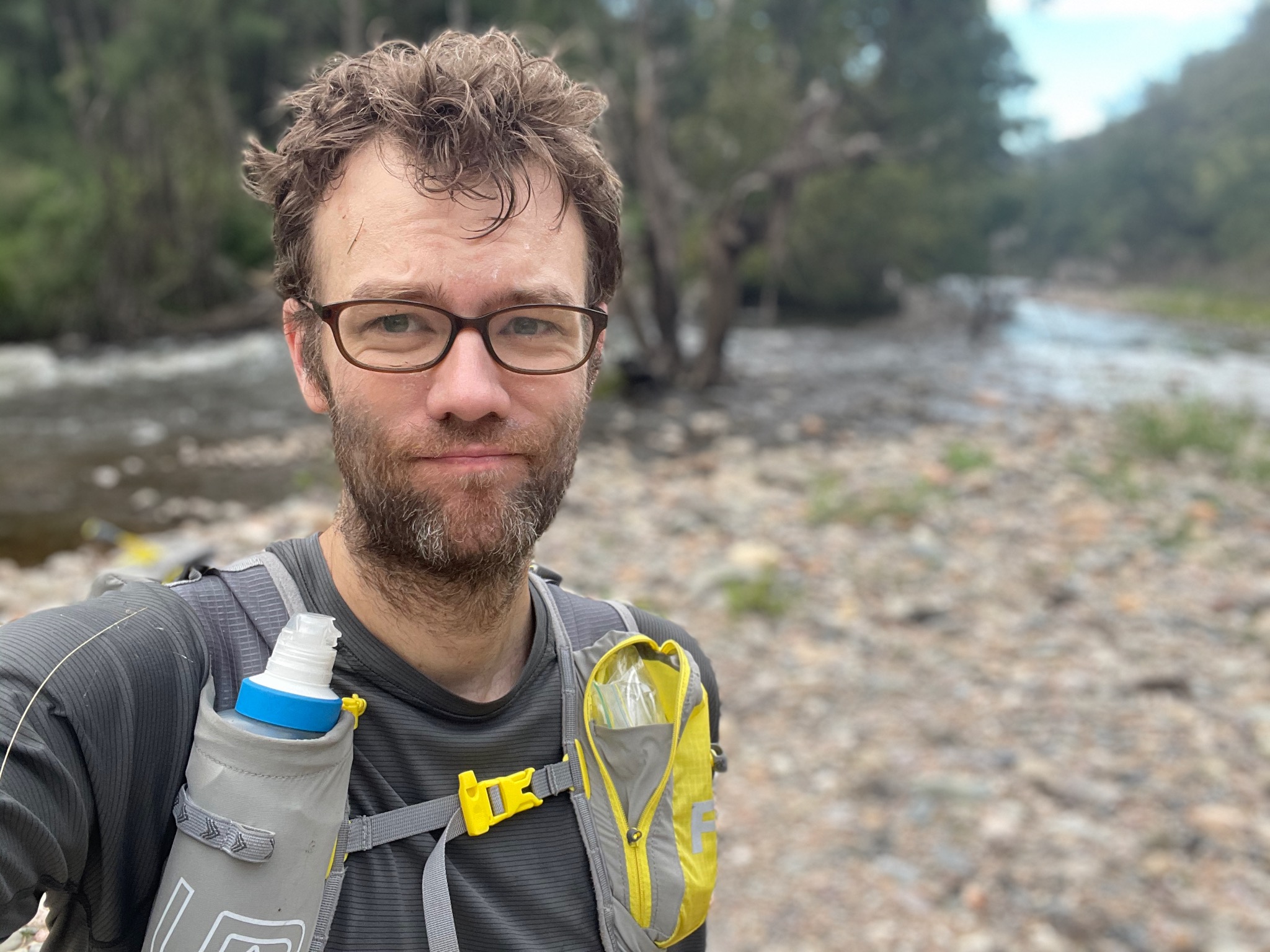
Not looking quite as fresh
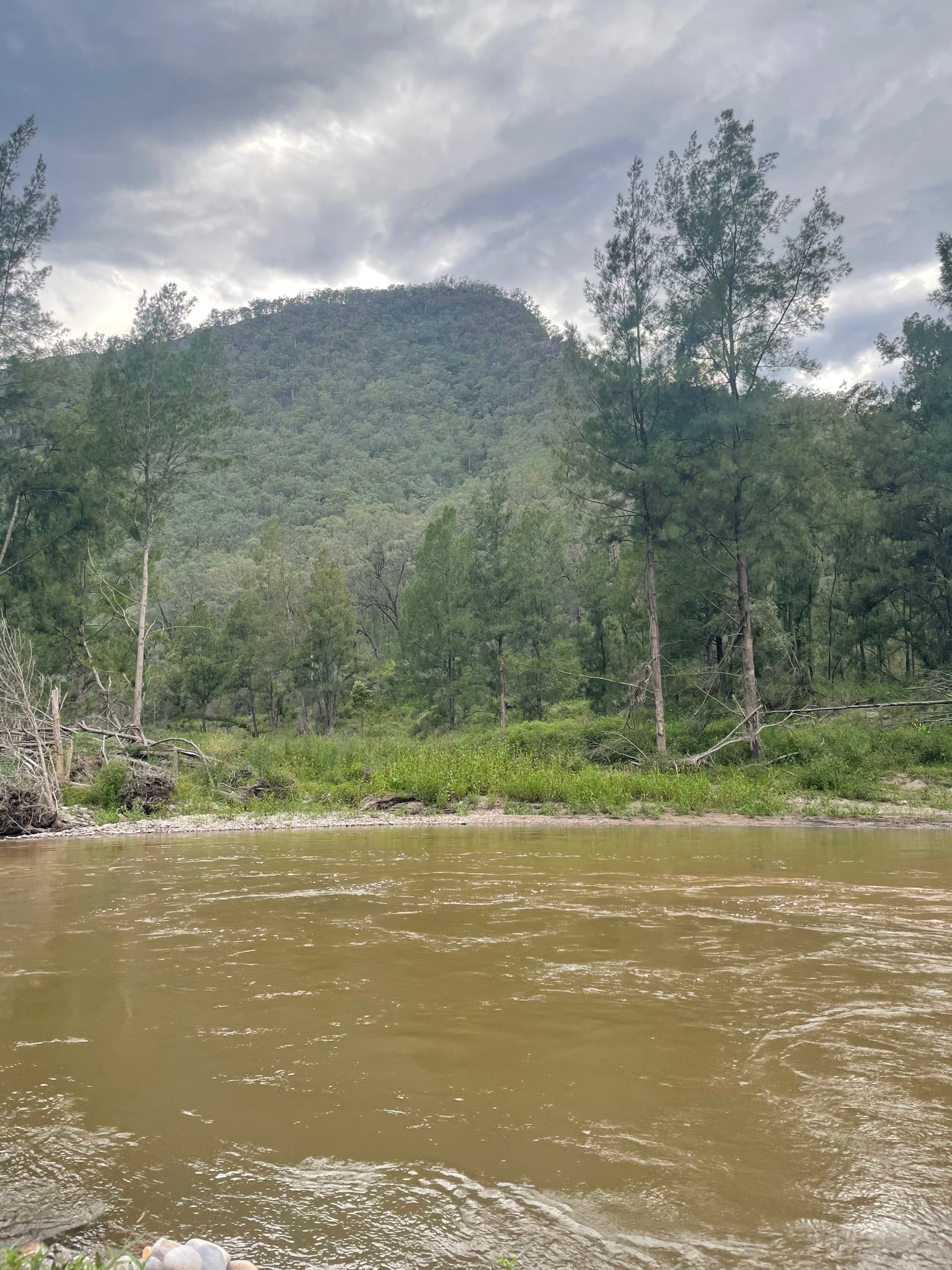
Too deep to cross, and need to climb up that mountain next
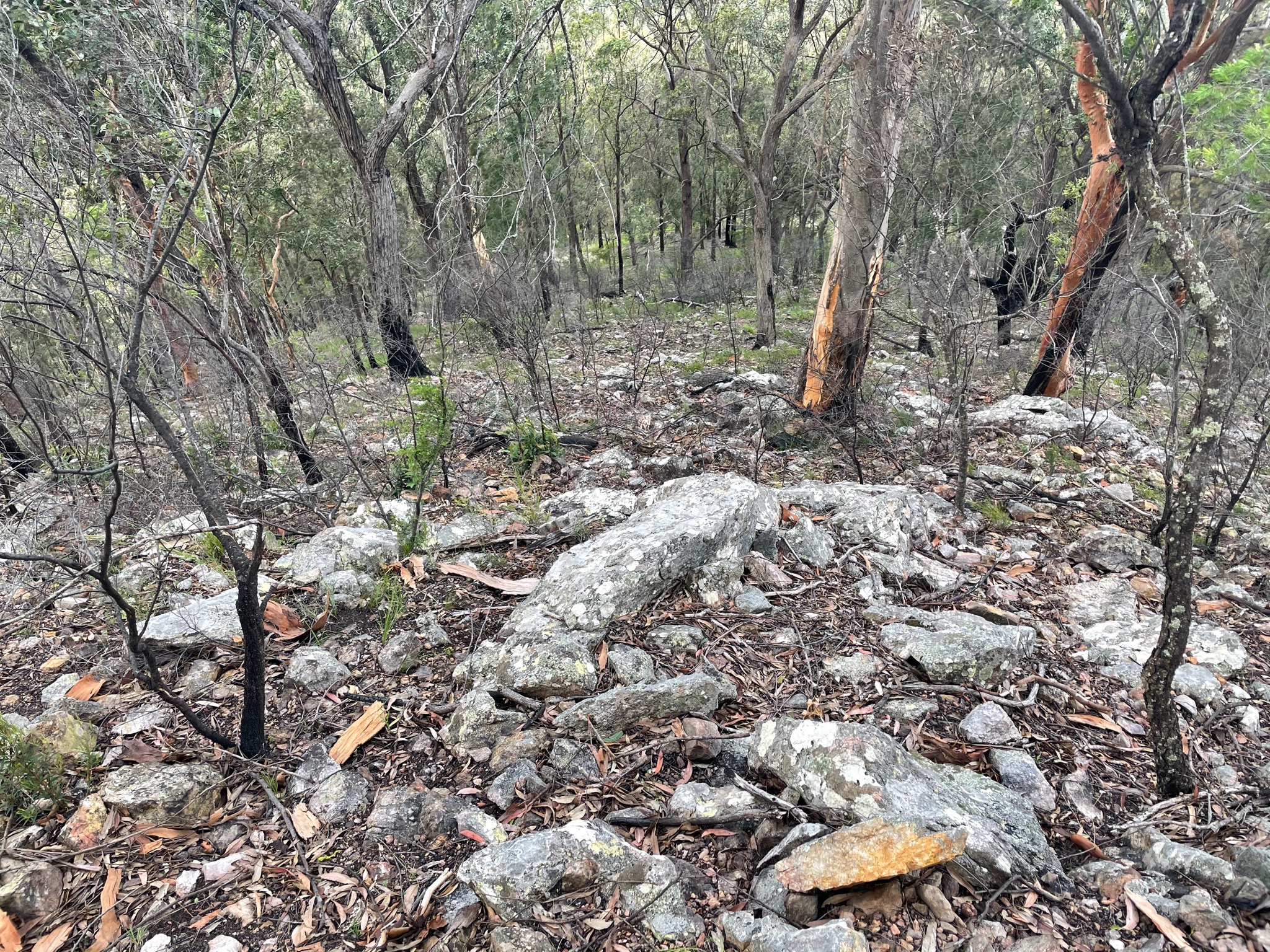
Much easier when out of the bush, but still pretty steep
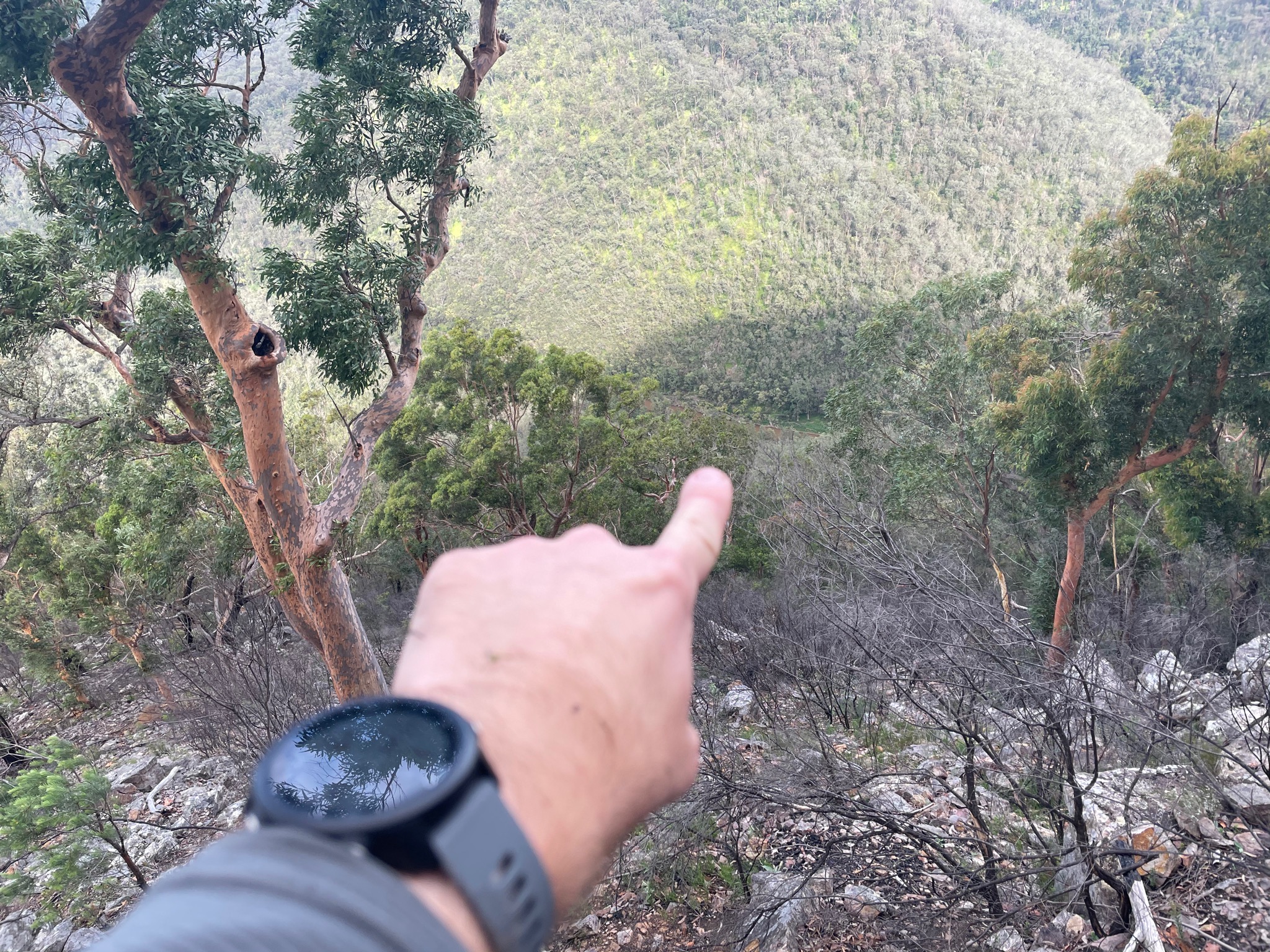
That’s where I came from
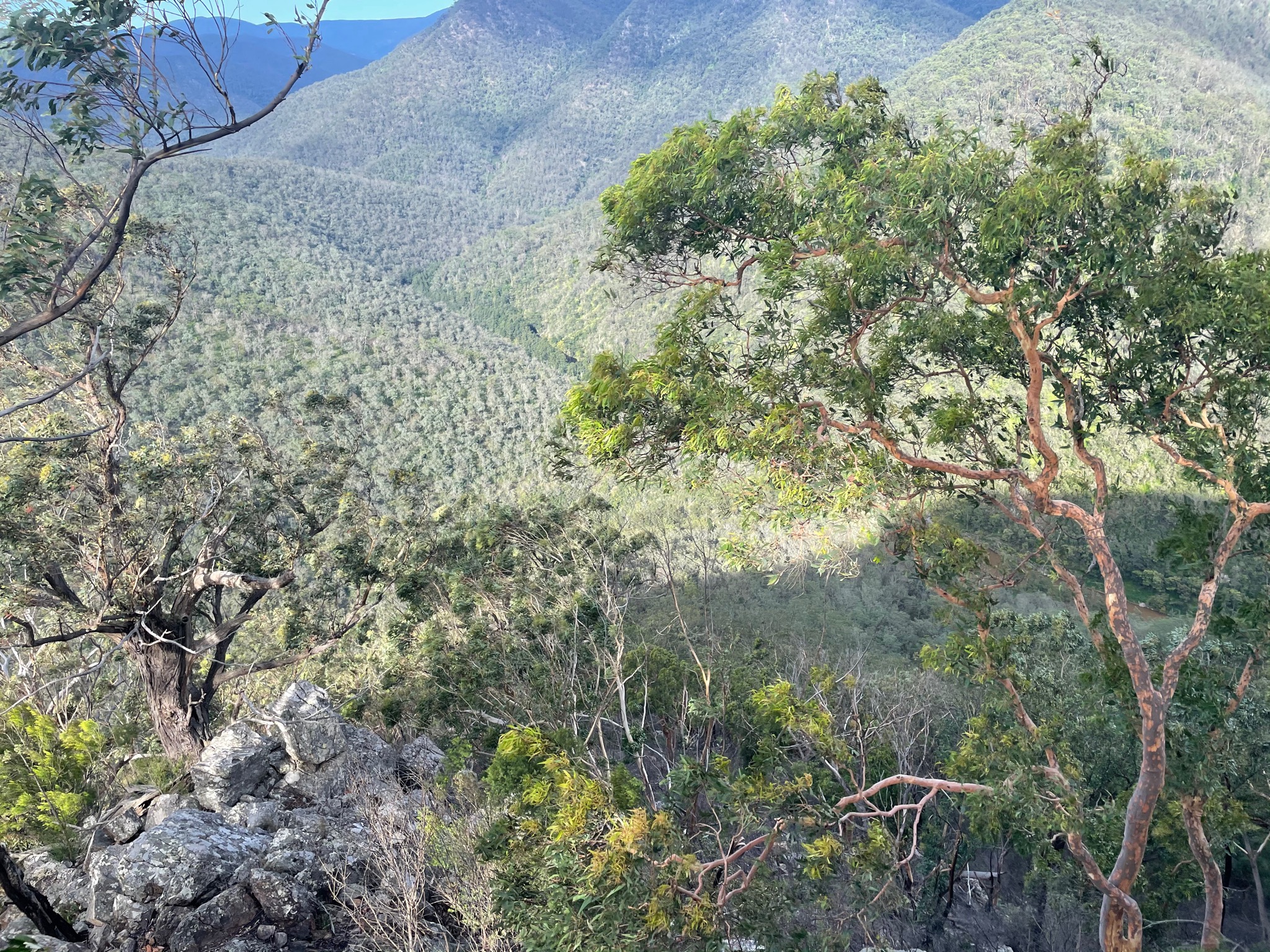
I think that green area is where I camped
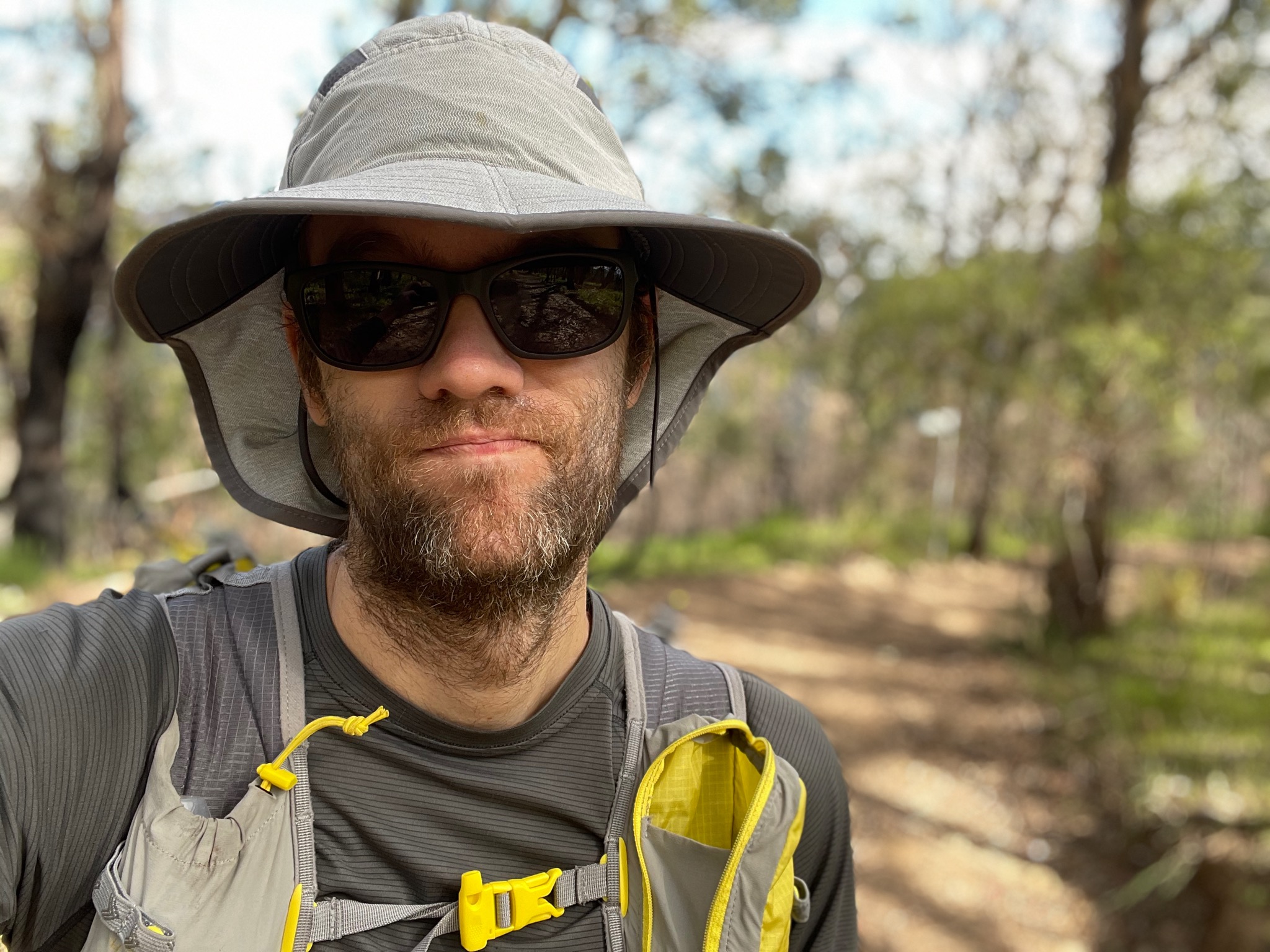
At least it isn’t raining
Day 2 (47km)
And here is where things start to really deviate from previous trip reports. The walk from Yerranderie to Wollondilly River was as per spec, except for needing to continue down Sheepwalk Track instead of my planned Roses Track, as it was closed to walkers. My heart sunk a bit when reaching Murrphys Crossing and seeing how big the river was - I was pretty certain there was going to be a need to swim across it, and it was flowing quickly. I sent a message on my inReach, and walked downstream to see if there were any branches and logs that I could get trapped in if I did get swept off my feet. I then started across, and luckily the water never went above my waist. There was good footing the entire way. Looking up the river was beautiful.
Some more fireroad later and a sign pointed in to the bush reading “Mount Beloon”. There was a faint trail, and a pretty easy initial “off track” experience, at least compared to bashing bush that’s as tall as I am. Soon the waist height bushes returned, and this time on the steep hill. At some point the track intersects the cliffs around Mount Beloon, and I thought “faaaaa I could maybe climb this, but in the rain this is going to be sketchy”. Keep looking around and eventually there will be an easier section that requires NO CLIMBING.
Coming down the other side I was excited to be going downhill, but the bush was now quite thick. Soon it dipped in to a dried up creek, which was a little faster to walk through. Continue down the gully, but be ready for a lot of scrambling. I’m not certain if the gully has always been like this, but I think it might have had a few landslides last year: trees and boulders were everywhere. It took about 90 minutes to get to the Nattai River.
One source stated in 2015 that “it was really interesting to see the wheels of time grinding down on the Nattai ‘Road’. Once upon a time it would have been used by 4WD, but now it is completely overgrown and impassable to anything and everyone who isn’t hiking.” Well, I couldn’t even find the road, despite looking, and I had offline maps and GPS. Looking down the Nattai, with no track or easy walking ahead, it was at this point that I knew I likely wouldn’t make it back as per plan.
I reached for my inReach and sent the preset “All is well, but behind schedule”.
Previous reports seem to indicate that the river bed is easy to walk along, but it might be necessary to cross the river a few times. Well, let me tell you, there were usually only two options: bash some very thick bush, or just walk up above-knee deep water. And to make matters worse, a lot of the sand was very damp, so I frequently would take a step and posthole knee deep in the sand. This postholing would sometimes go on for over 50 metres, and was very slow going.
The sun set and I was now hiking by headlamp, but with absolutely no suitable campsites visible, I was getting a little worried. Finally a small patch appeared, and I pulled out my gear and went to bed.
11hr 41min of moving time, but 14hr 55min elapsed time
Strava: https://www.strava.com/activities/4536901043
Photos: https://imgur.com/a/L2Sf7UJ
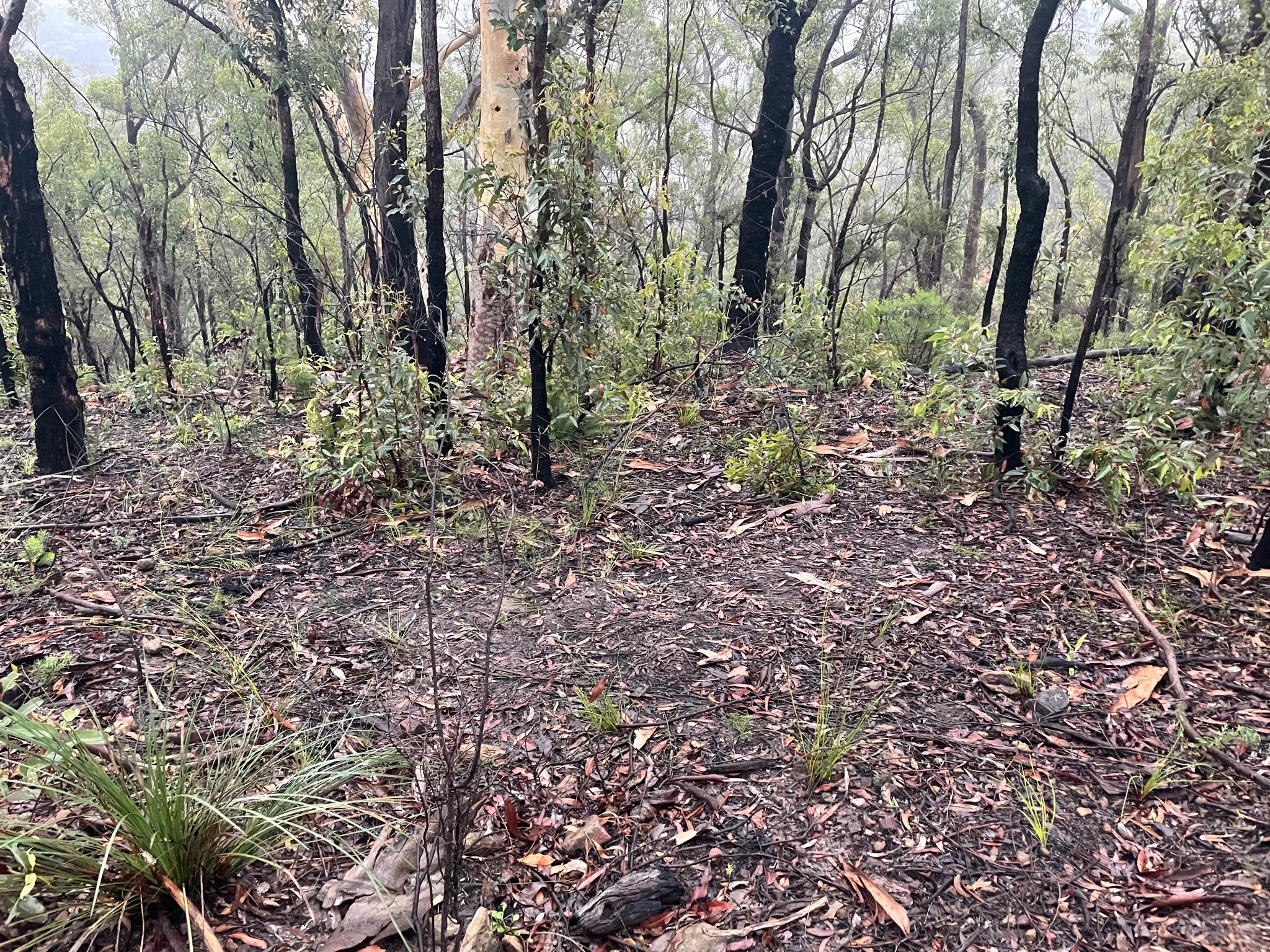
That’s where I slept
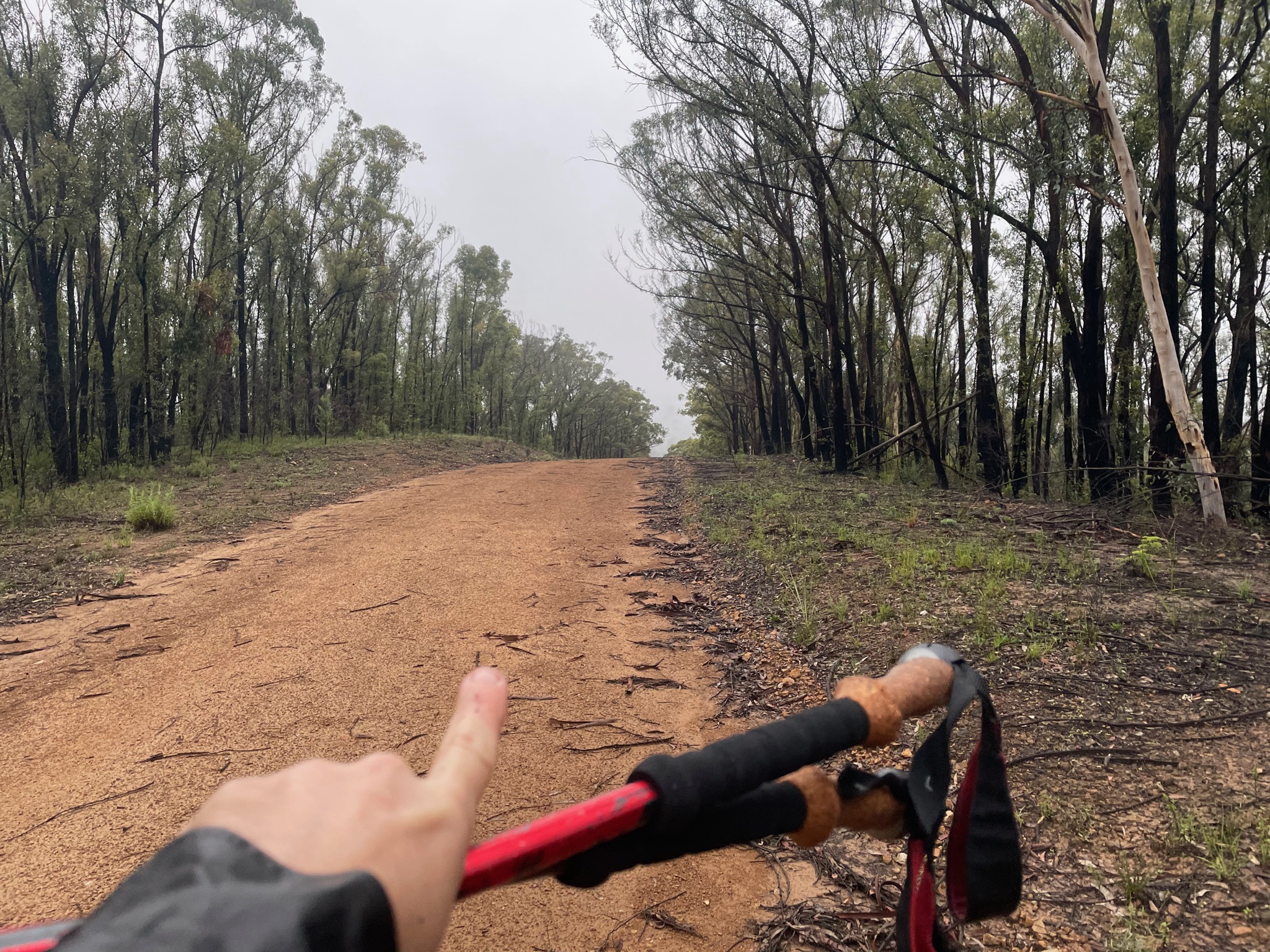
That way!
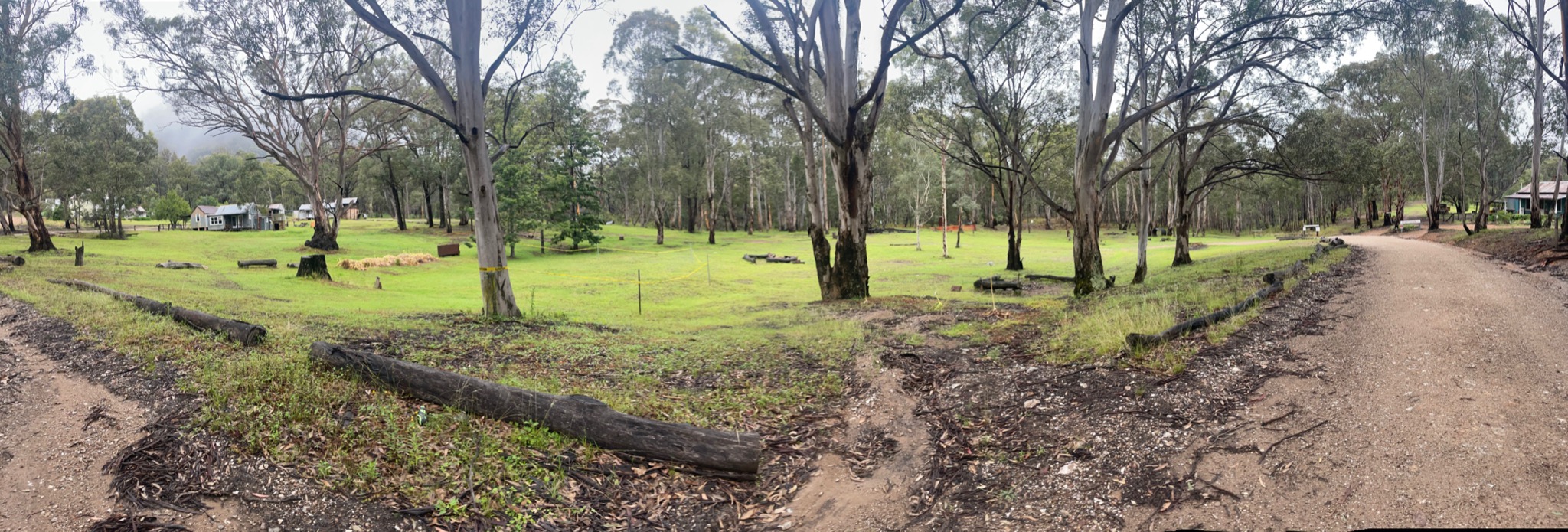
Under maintenance
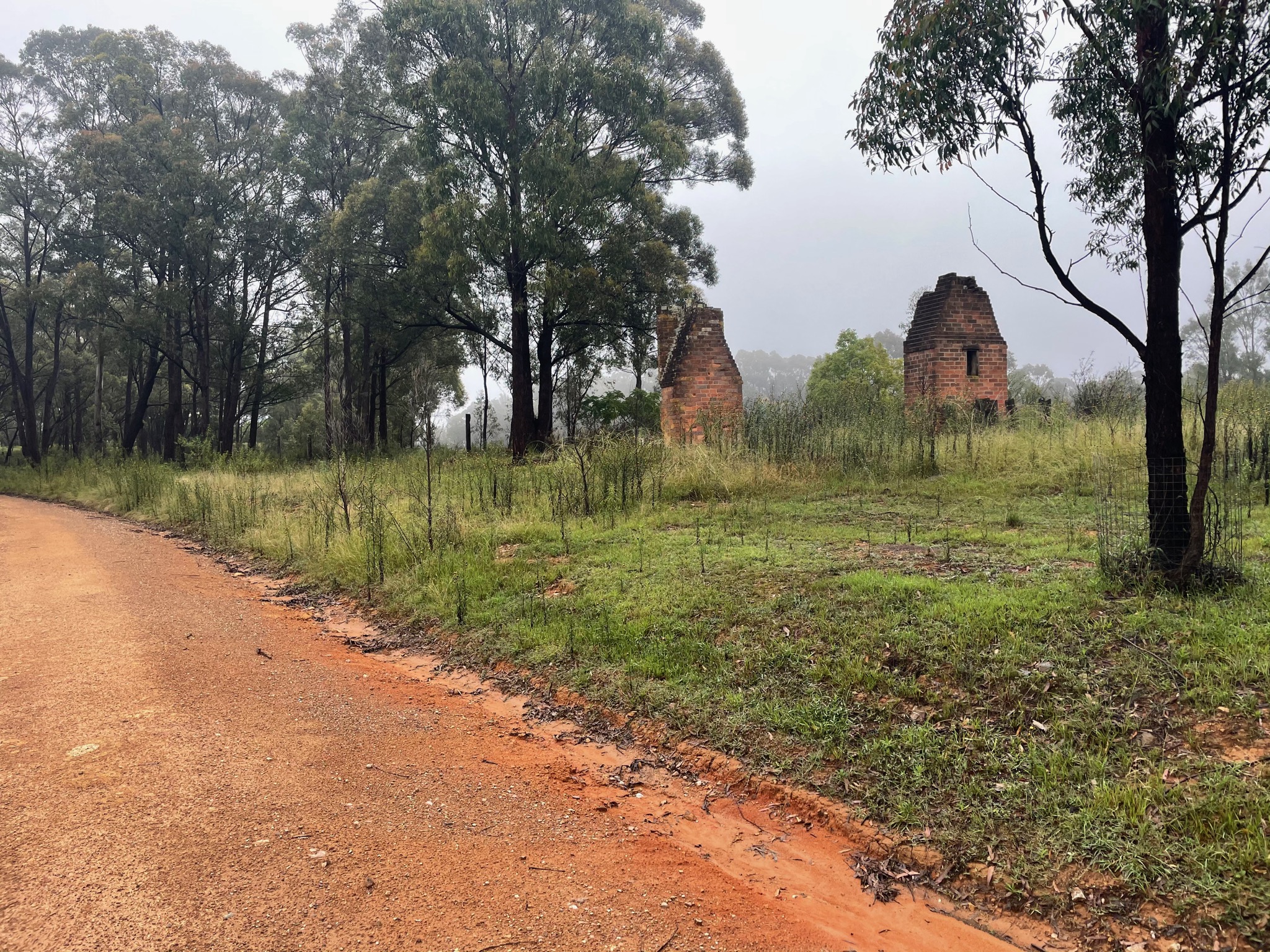
Yerranderie (Under maintenance this week)
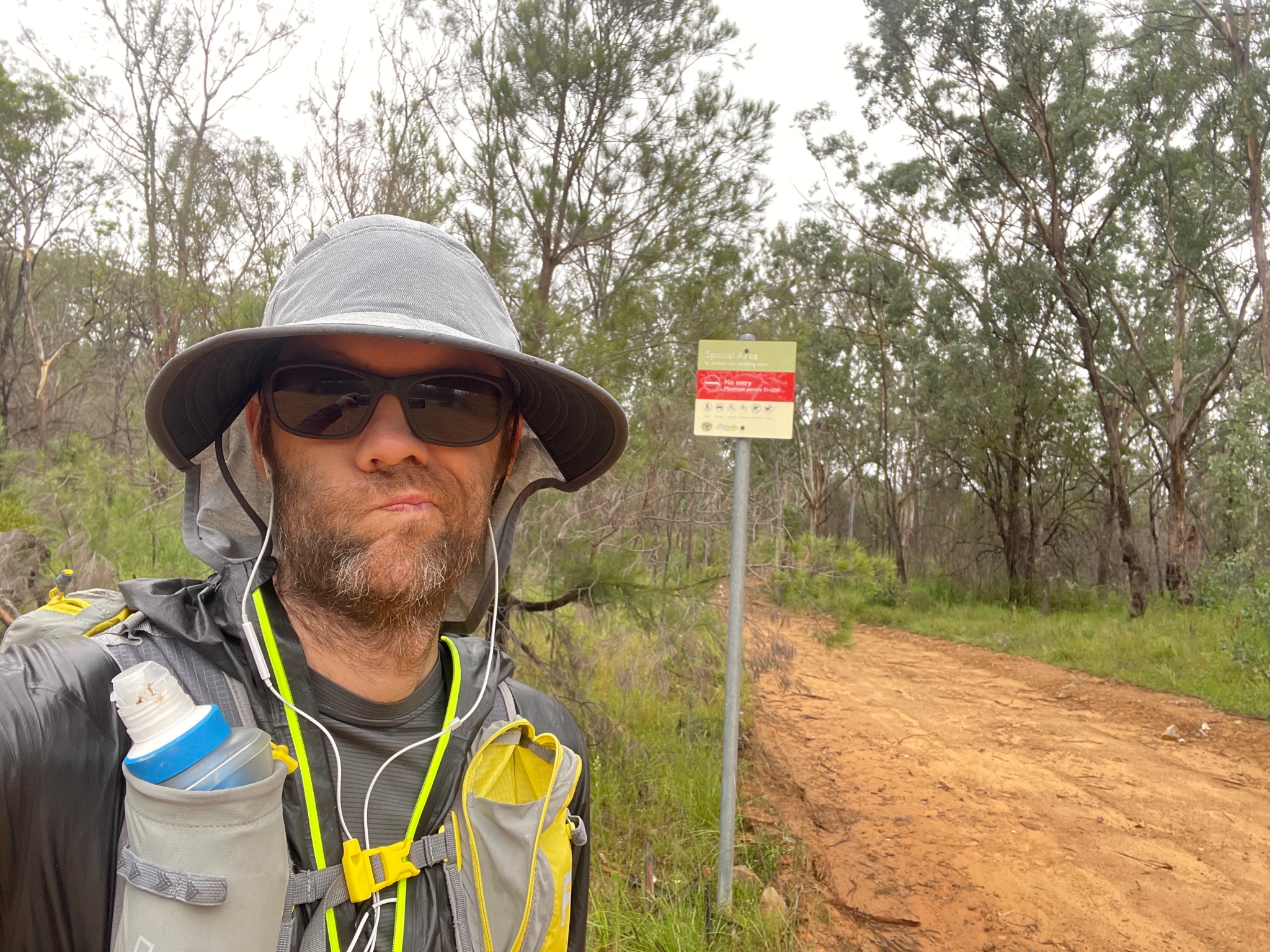
Nope, not going to go that way
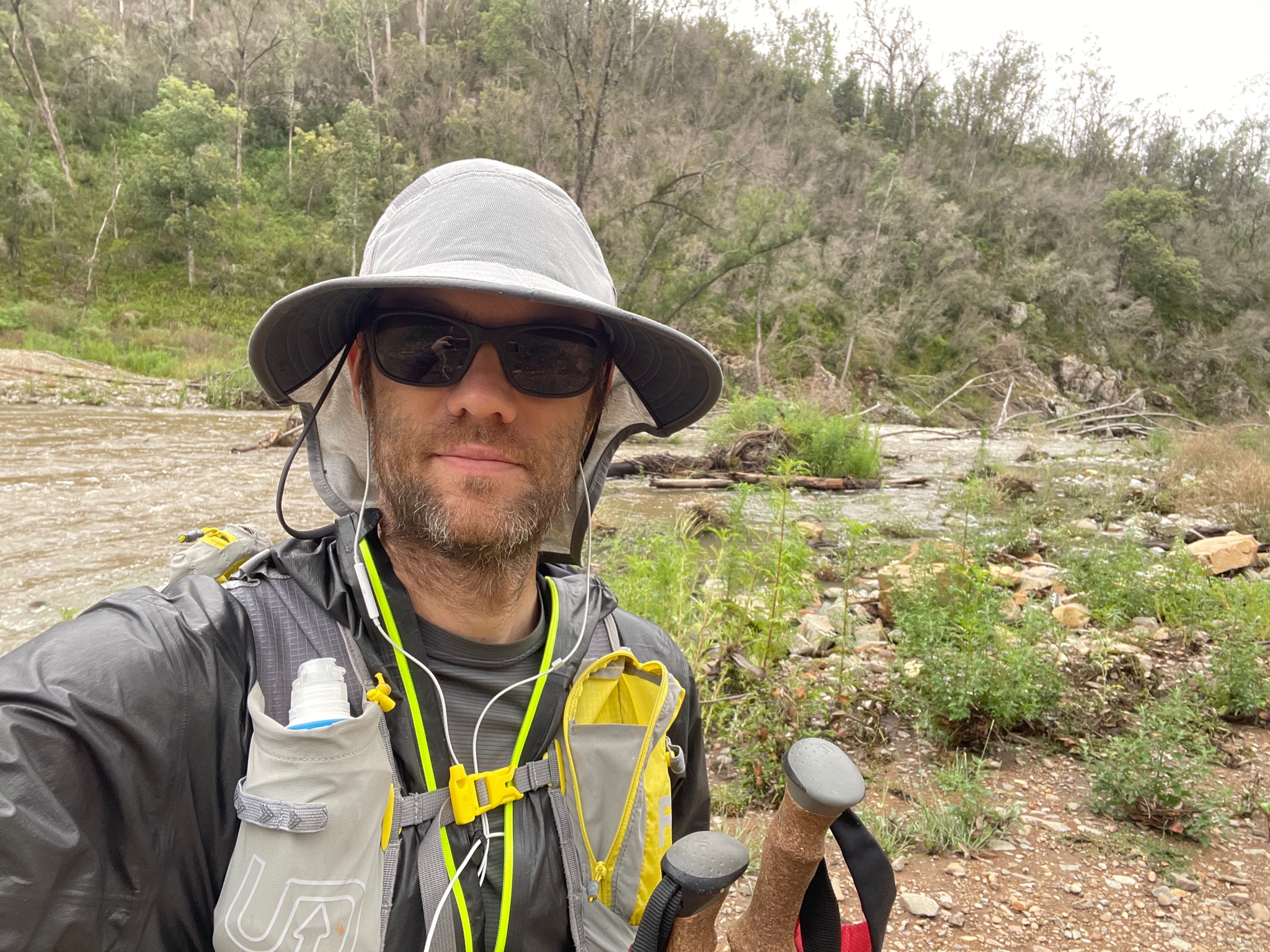
Spirits still high
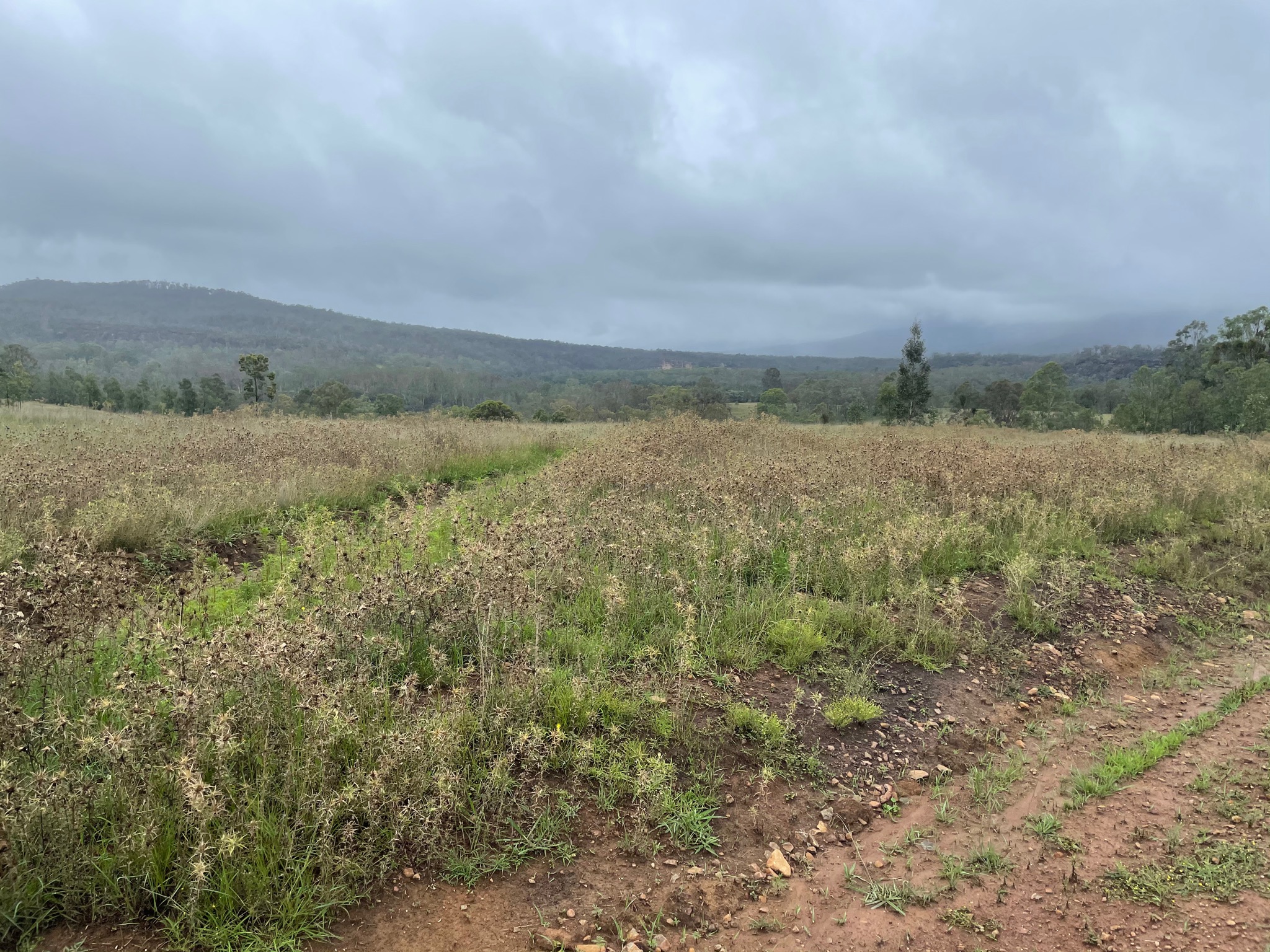
Beautiful remote views. Lots of roos and emus
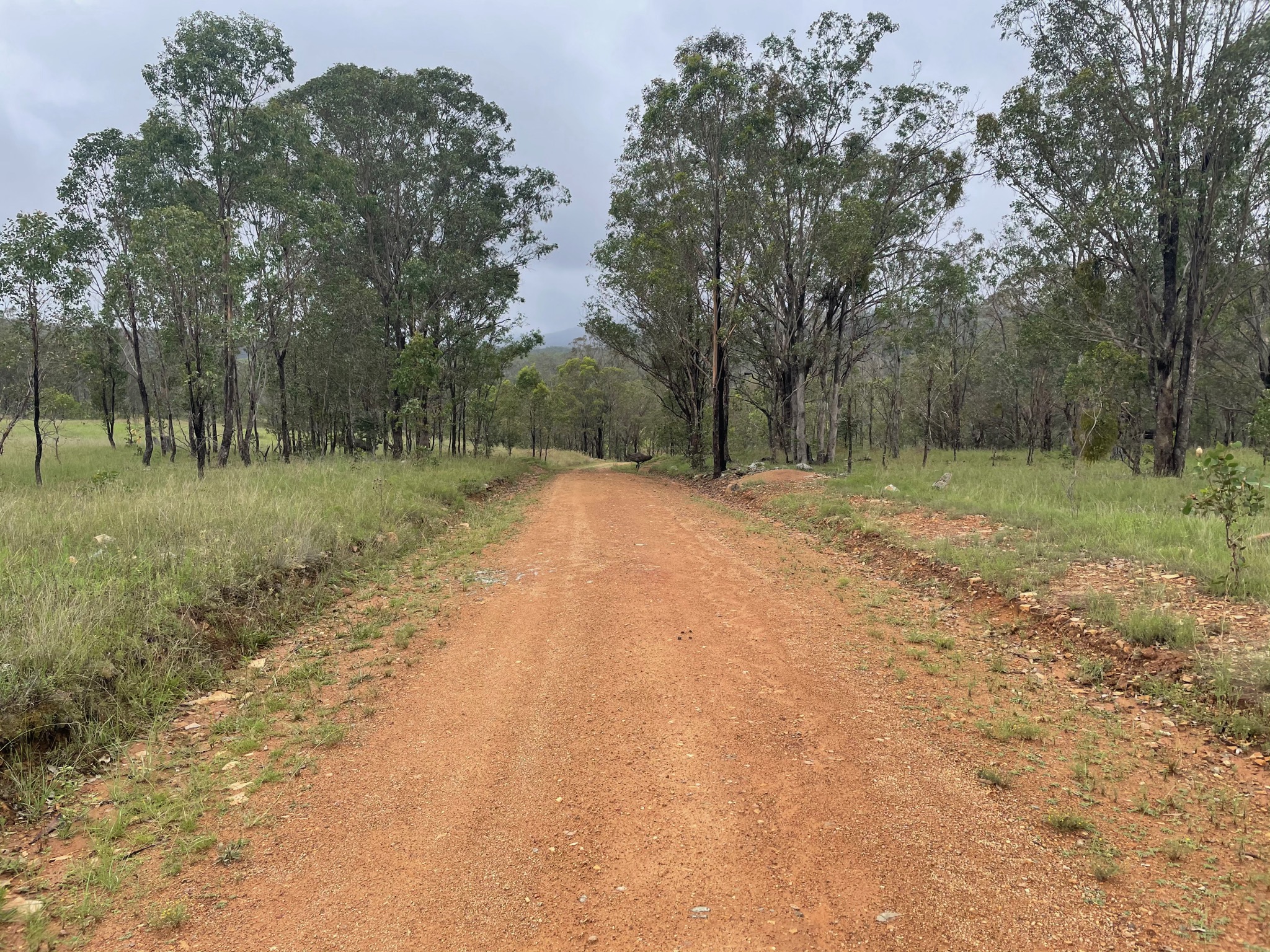
One of my favourite stretches of road
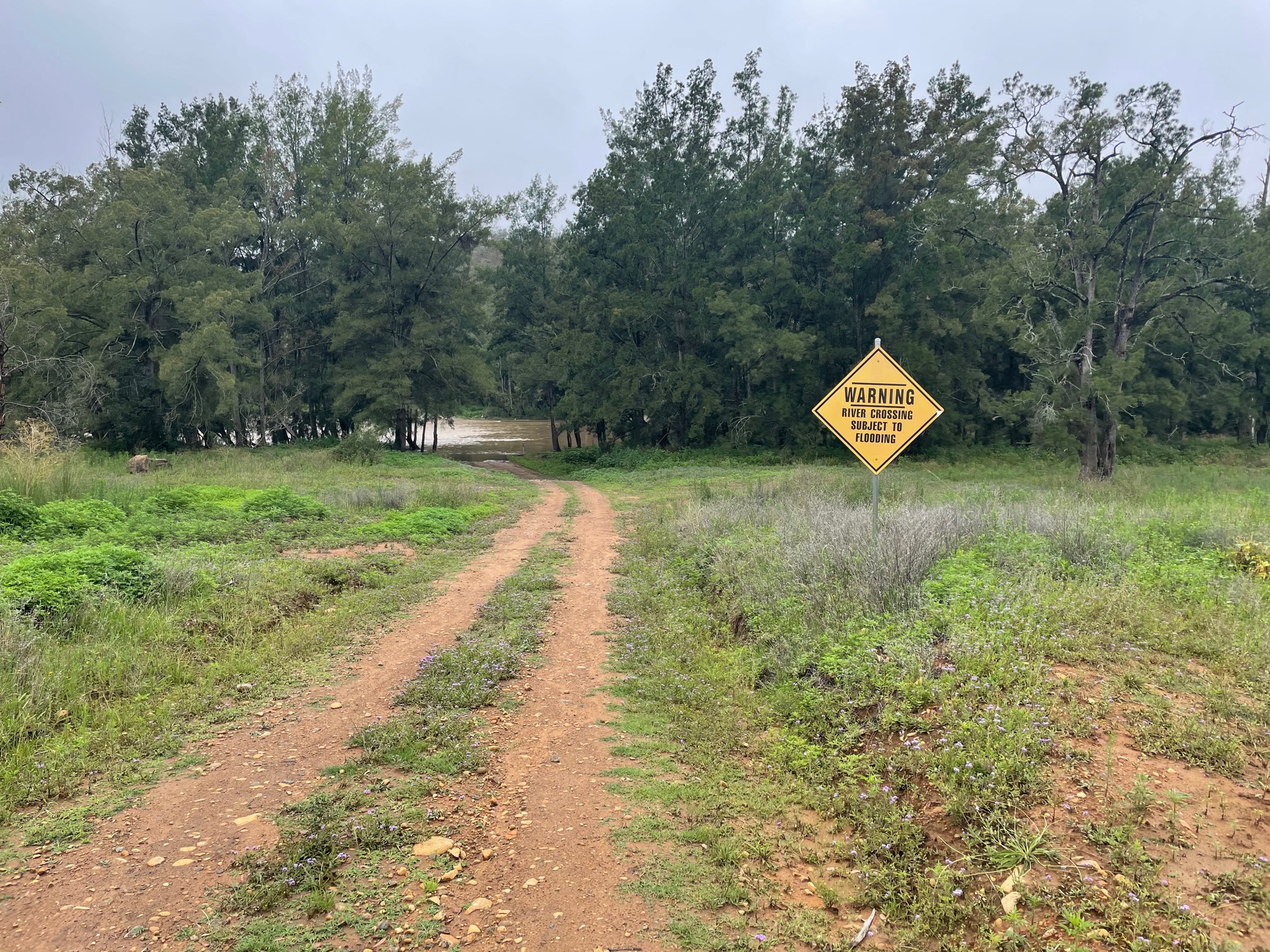
May be subject to flooding. You don’t say.
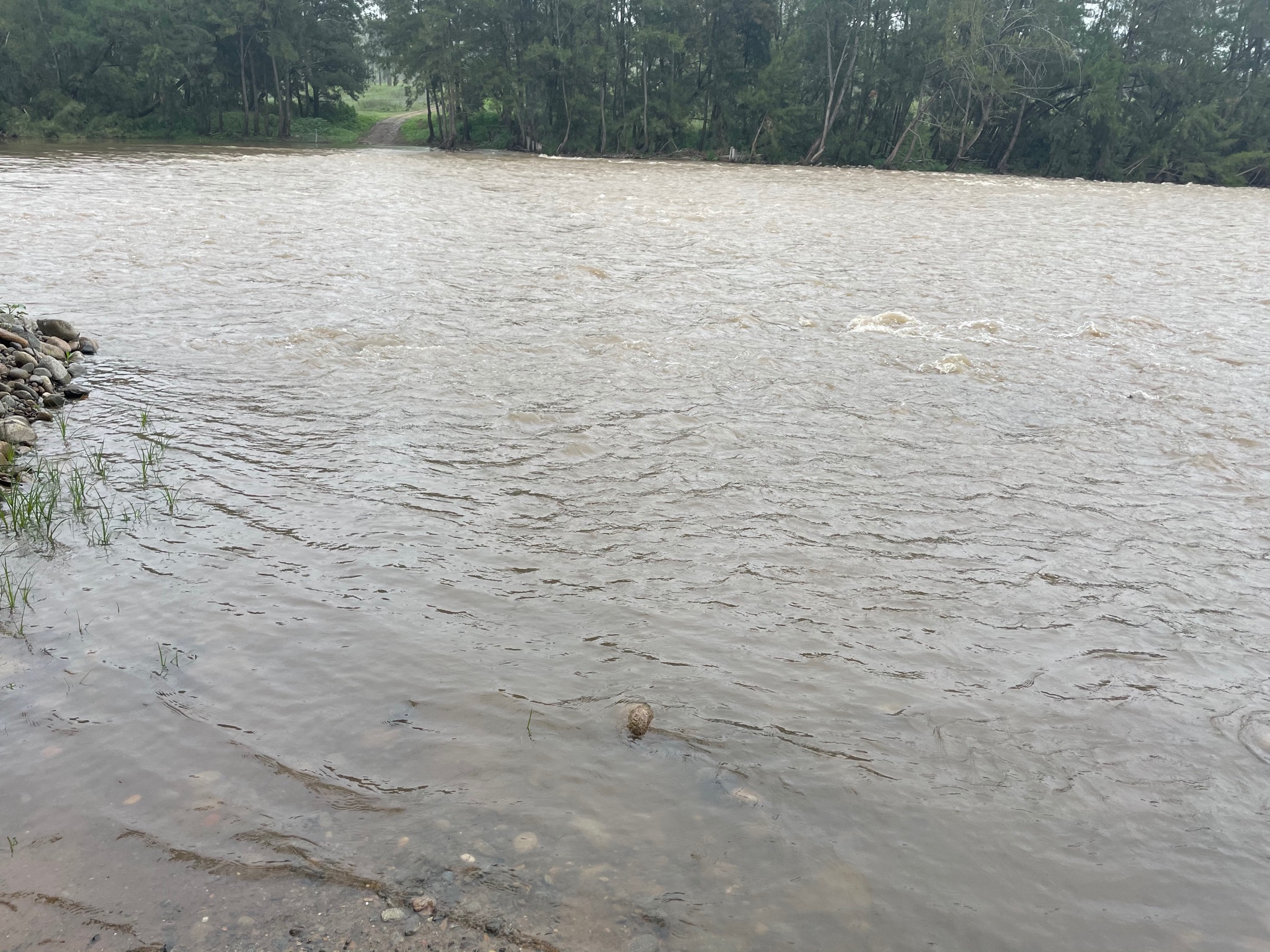
Survived.
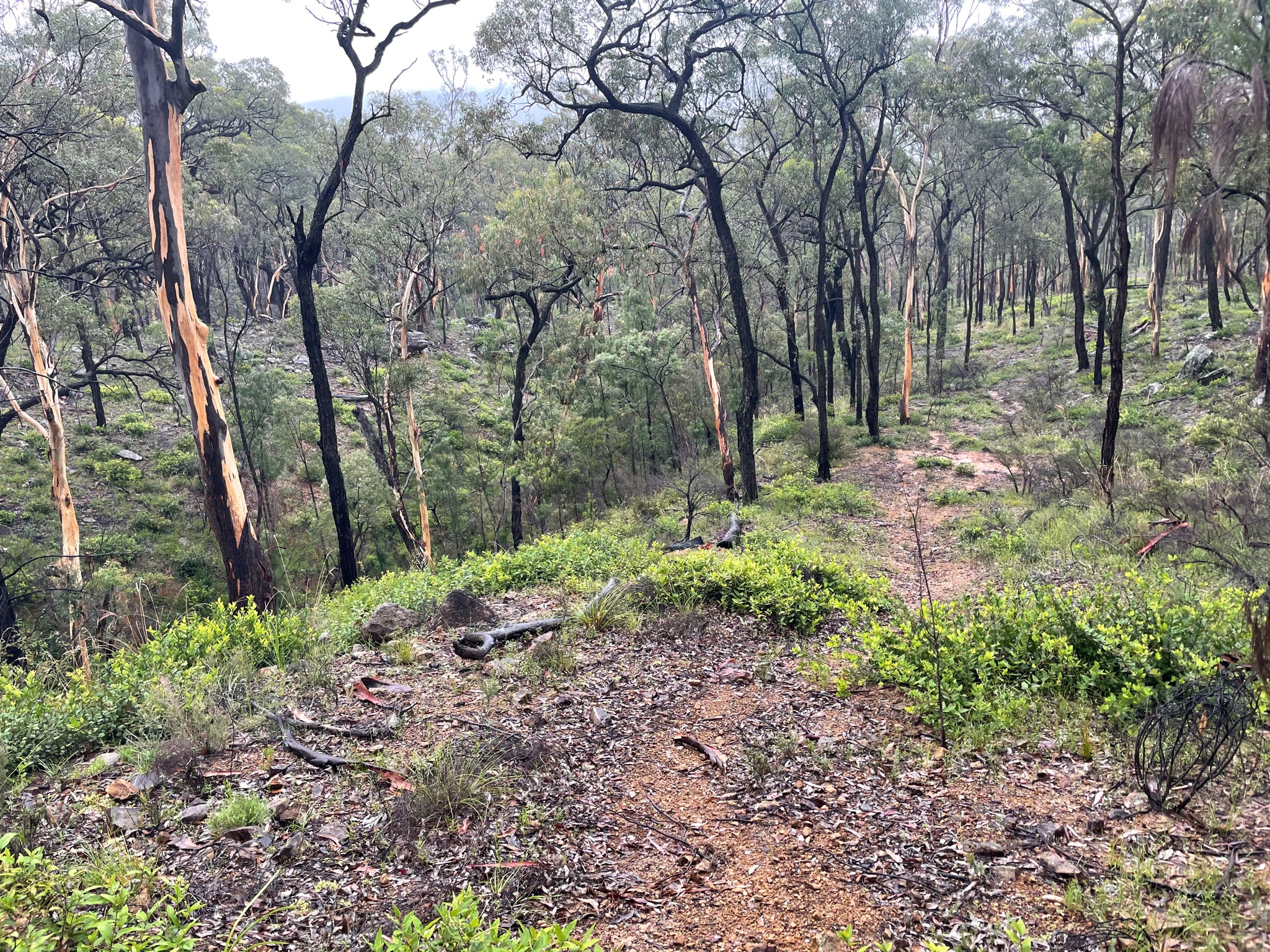
Easy off track
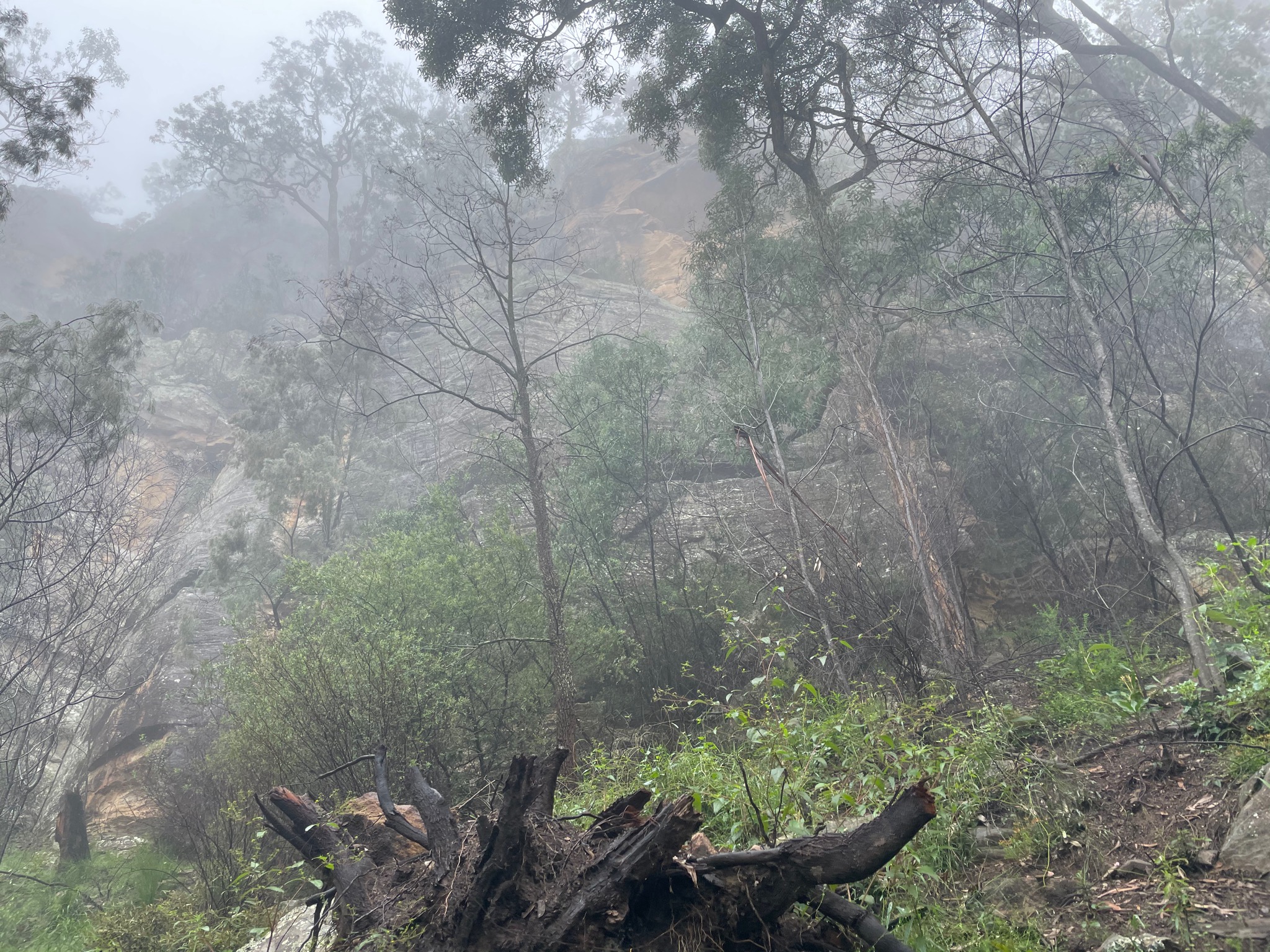
That’s a problem
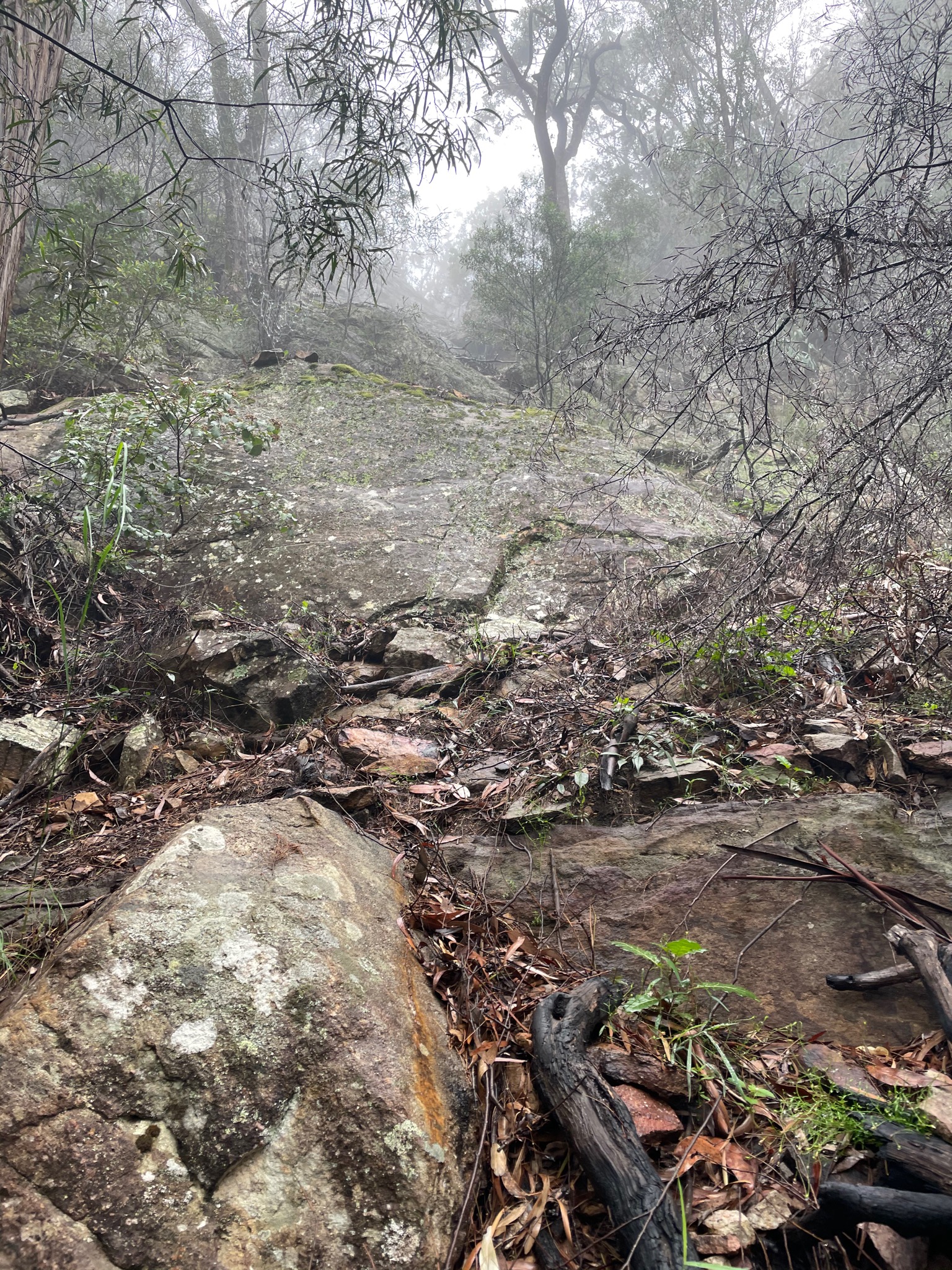
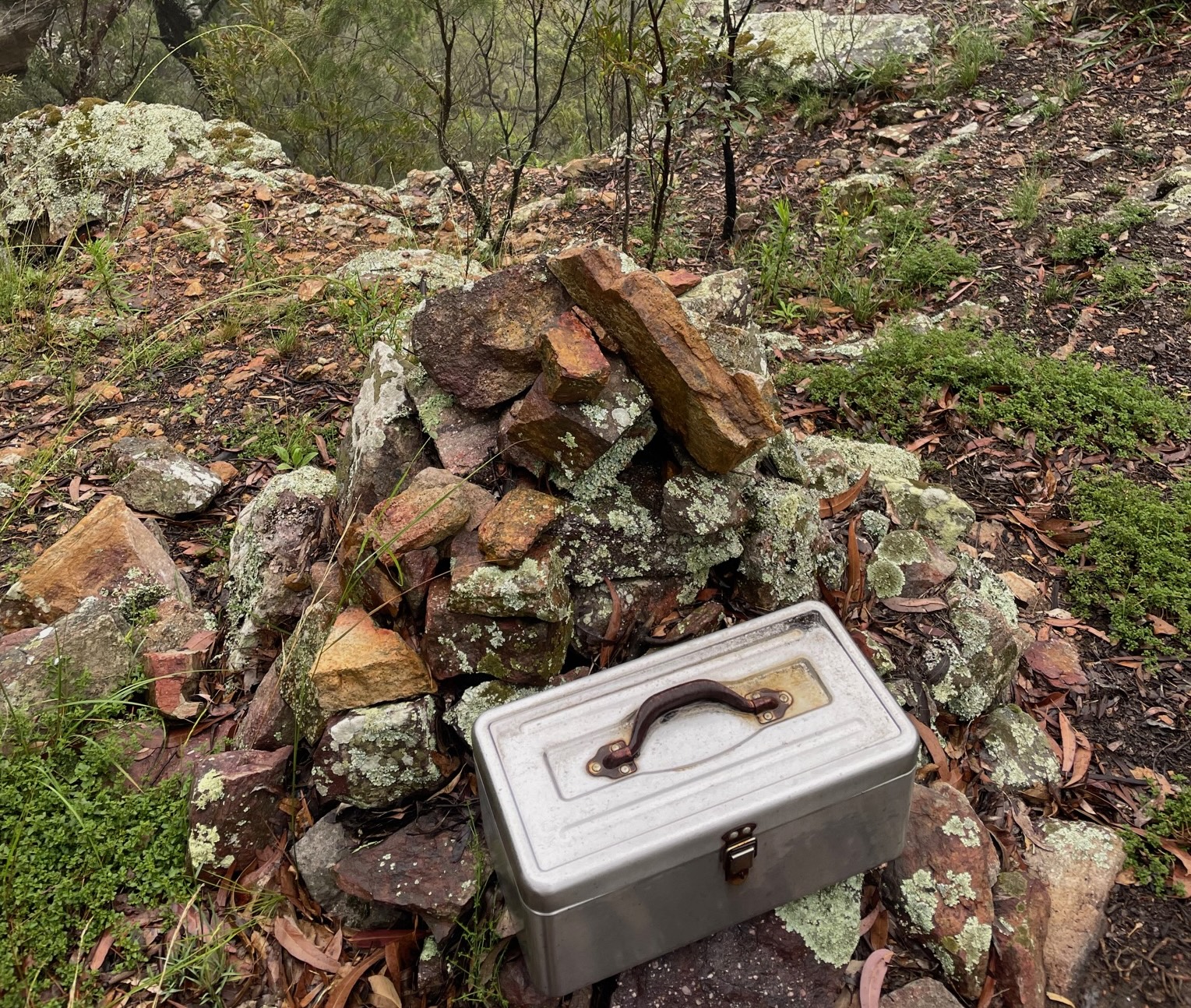
Made it
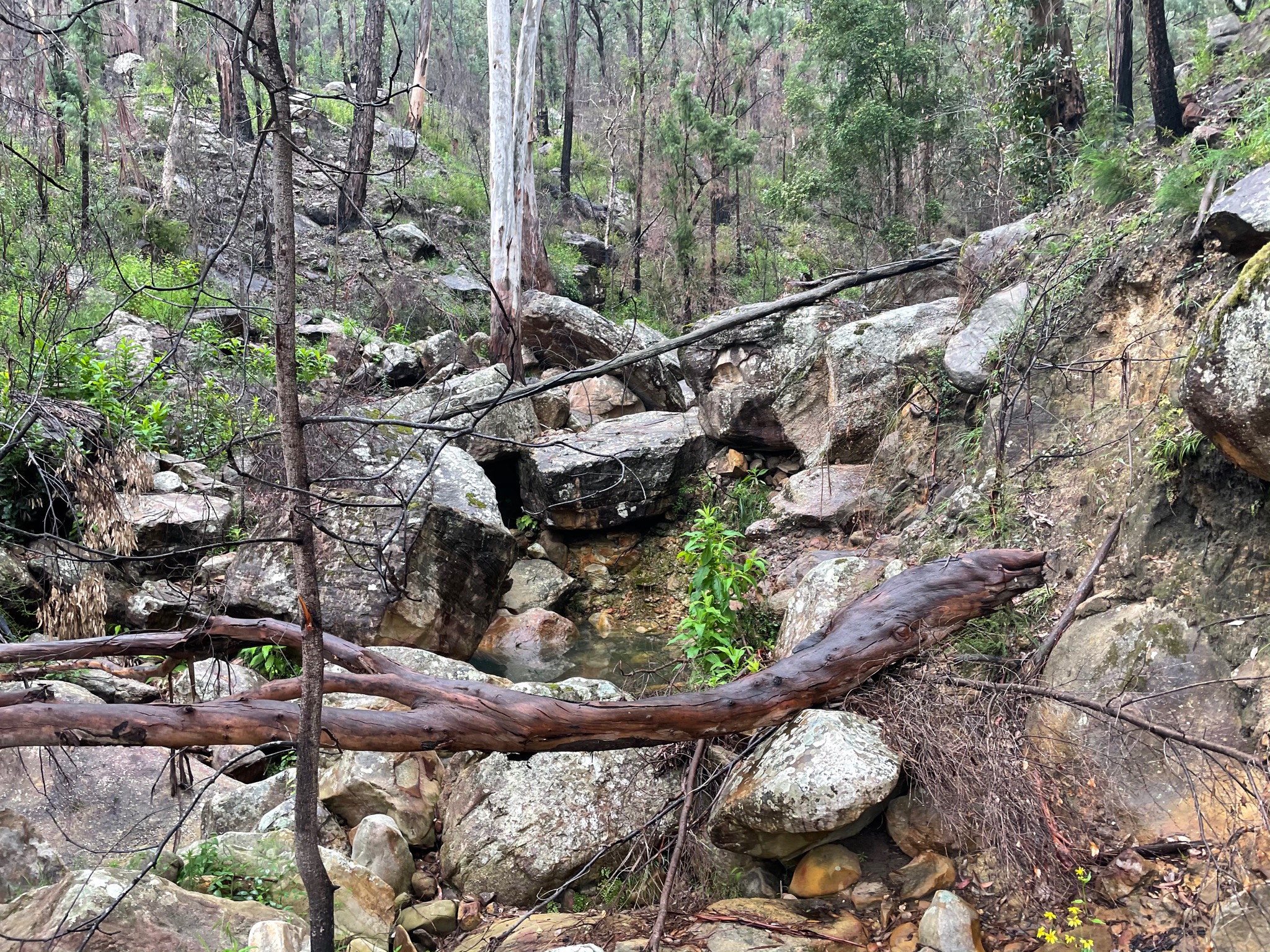
Only took 90 minutes to get down the gully, but a lot of effort
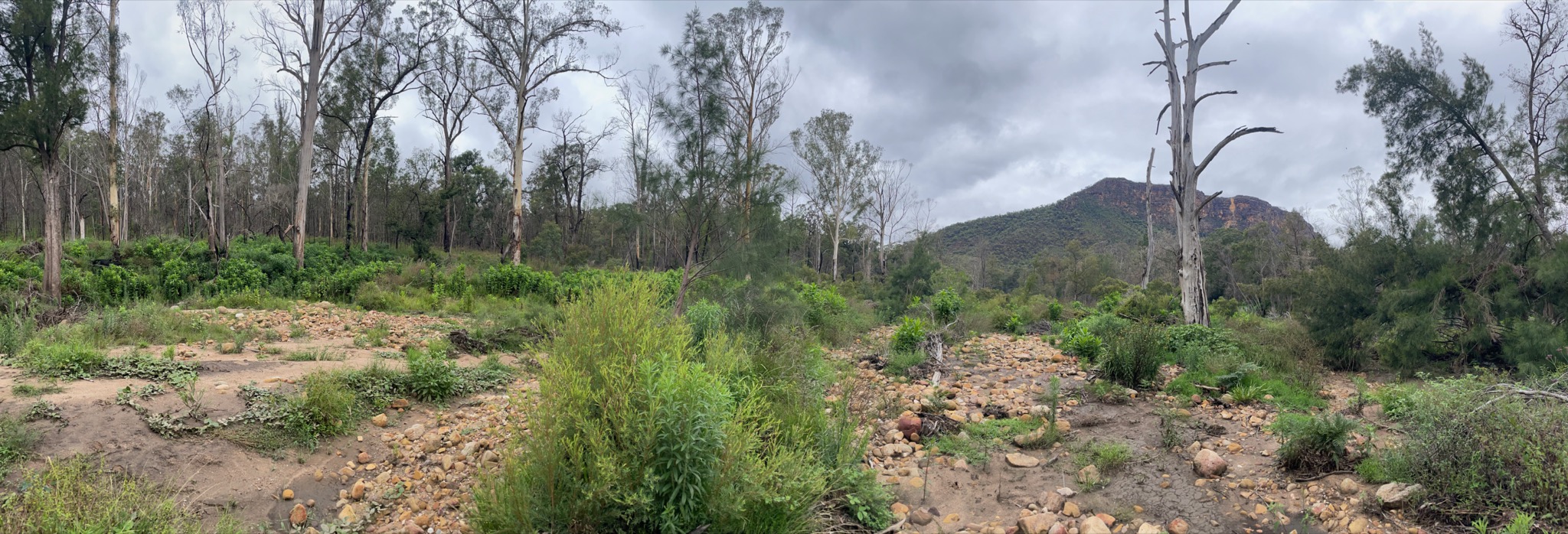
Nattai river
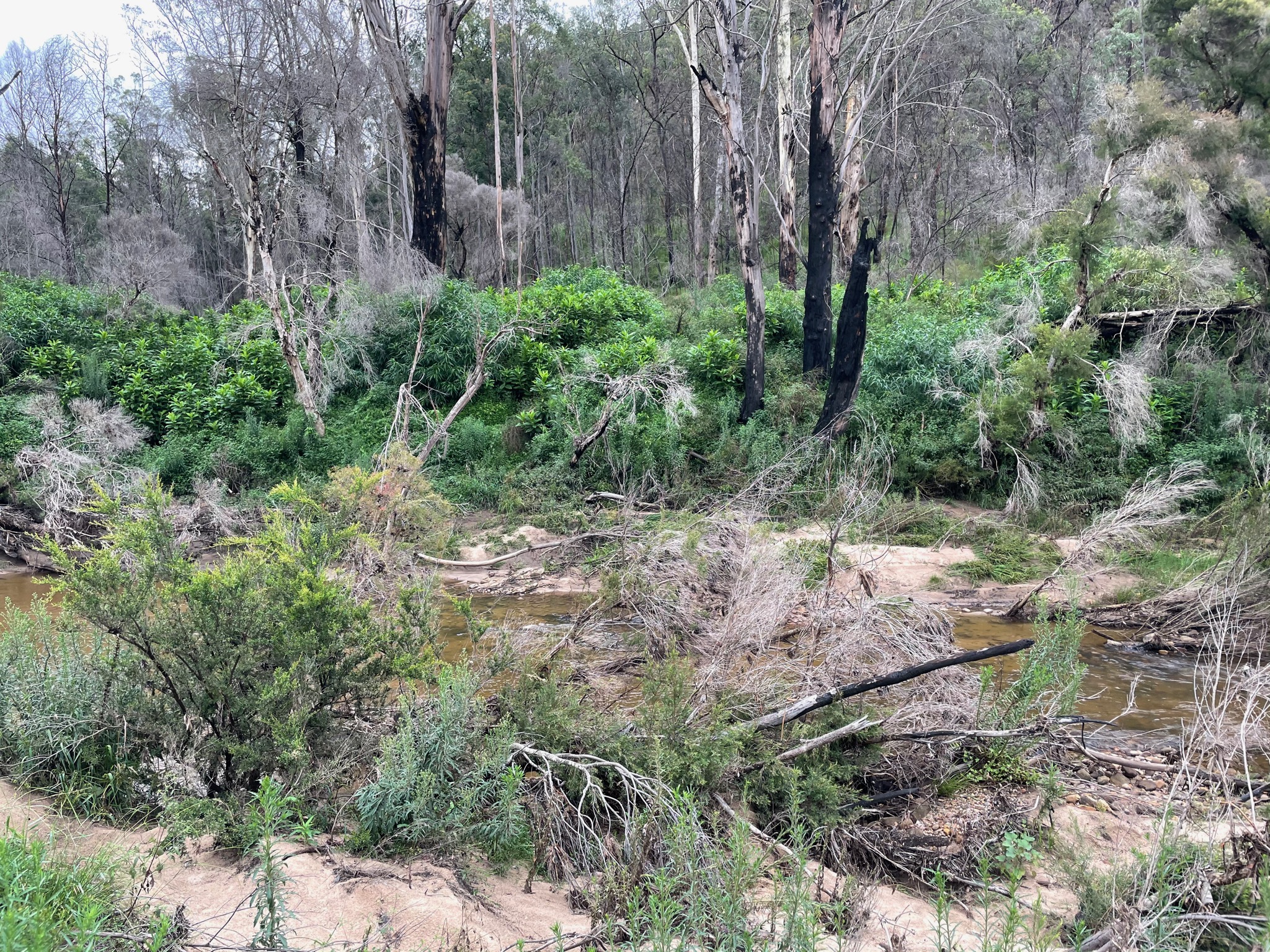
Bush whacking time
Day 3 (21km)
By 6am I was already packed and continuing down the Nattai, yet slowness persisted - maybe 18:00/km. Troys Creek Track was supposed to come out via Troys Creek, but I couldn’t find any sign of a track. Next I came across Emmetts Flat and started up the creek, but there was no evidence of human activity. And then I saw a cairn. Just two stones, but hard to miss. My spirits have never been lifted so much.
I found Starlights Trail, and was elated. I bounded up to Point Hill where I had the first mobile phone receptions since leaving Narrowneck, and out to Wattle Ridge. By now it was about 9:30am. The next challenge was how to get to Mittagong Station. I checked Uber (reported about $50), but no cars available. Then I checked 13cabs, but outside coverage. Because I arrived in the morning everybody in the carpark was coming in to the park, and not going out. I started to walk. I ended up walking almost the entire way to Hill Top, and not a single car passed me. Then one white SUV came, I tried to get a ride, but they carried on. Fair enough. A second car came, and they slowed down and picked me up! “Yea, I can drop you off at Mittagong, I’m going that way”. Thank you Jason - lifesaver!
Strava: https://www.strava.com/activities/4536954800
Photos: https://imgur.com/a/Ul2yLJ7
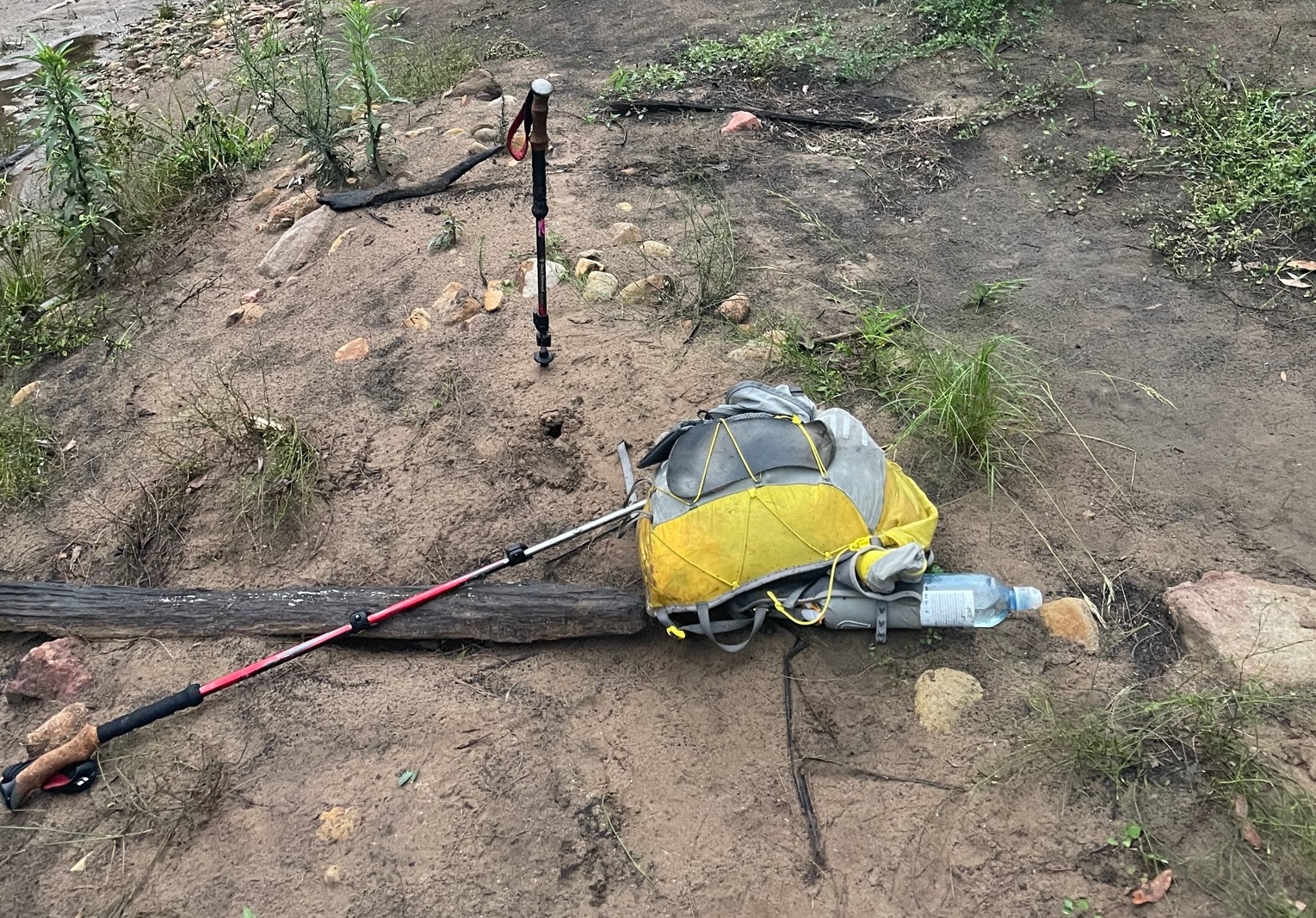
That’s not a real trail - wombat tracks.
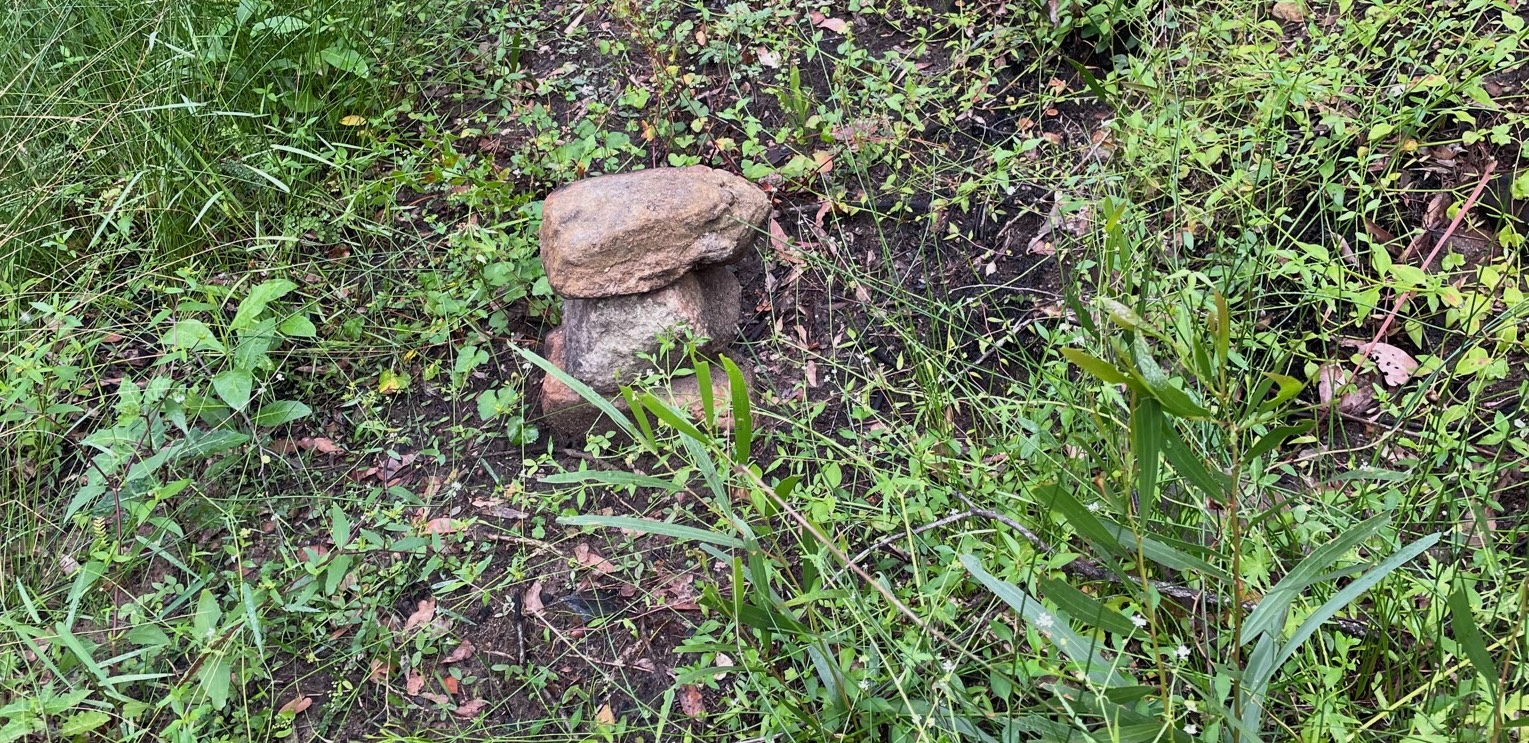
CIVILISATION!!!!
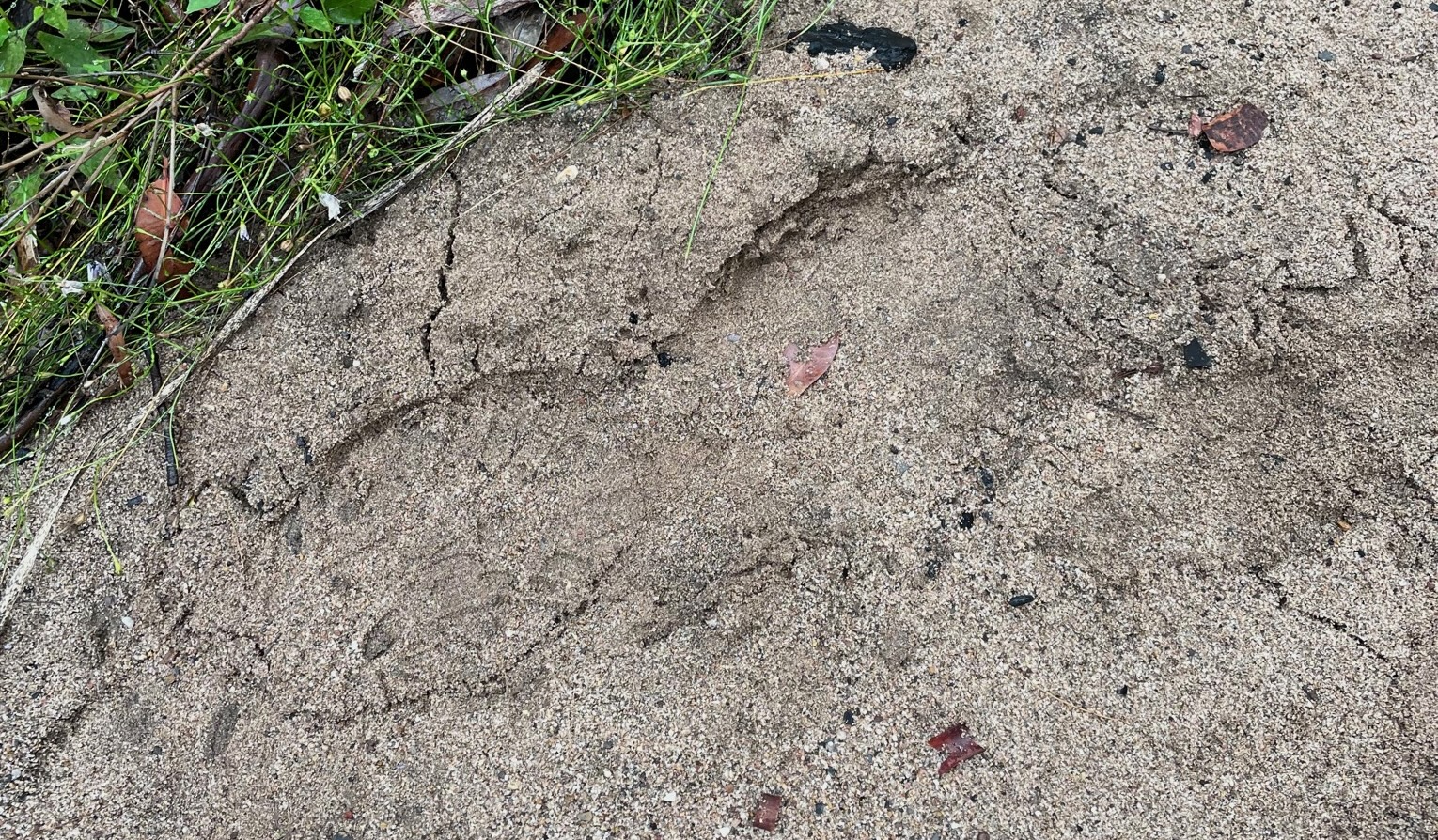
MORE CIVILISATION!!!
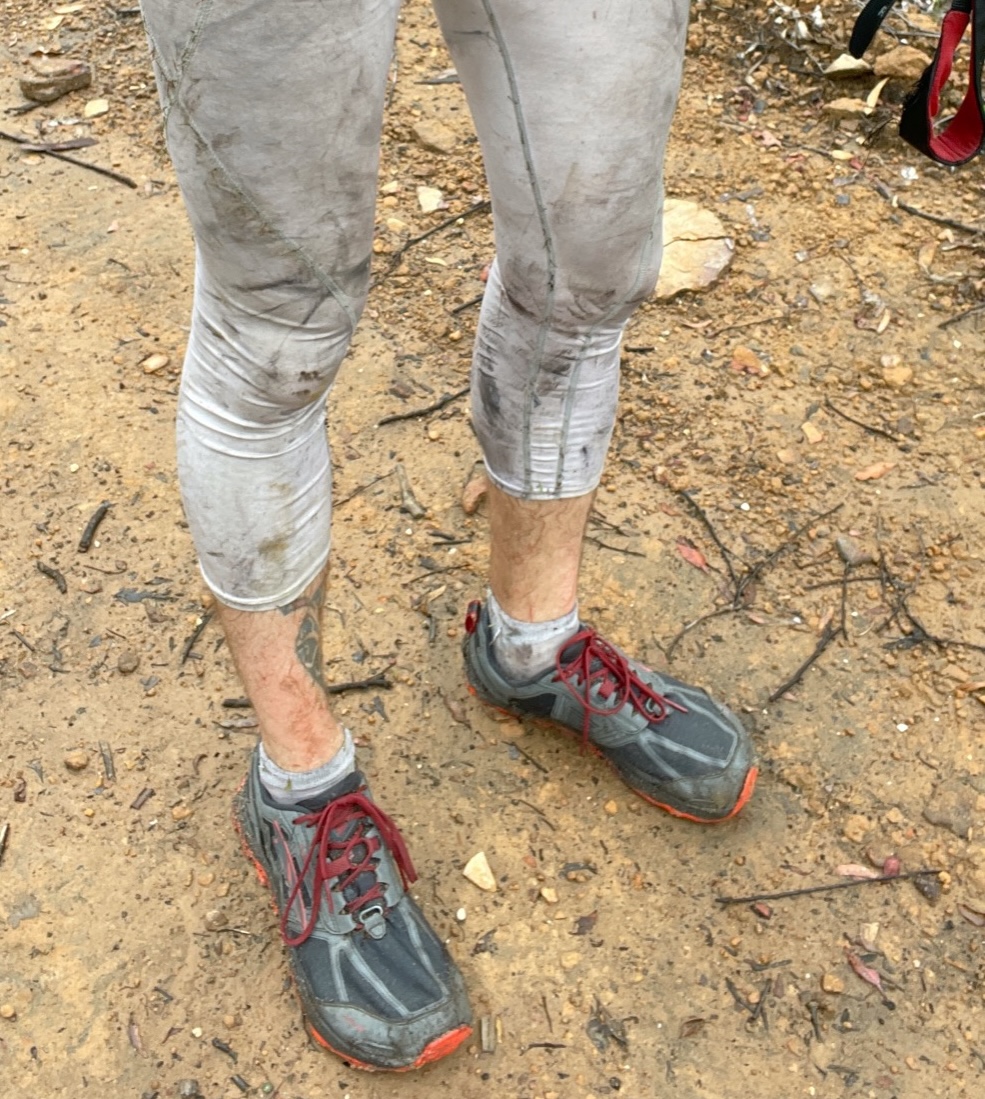
No longer see through
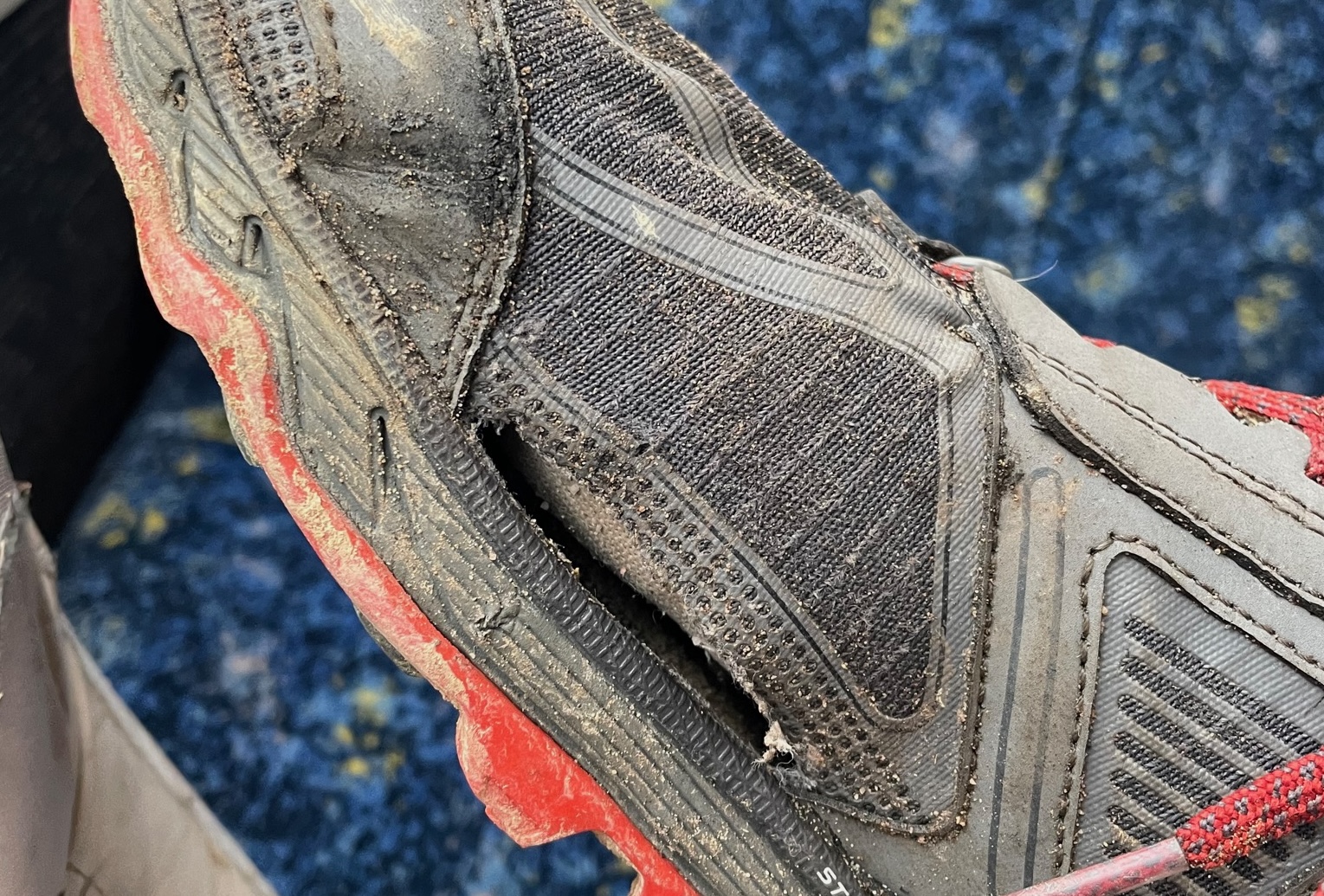
Gear malfunction
Gear Notes: I didn’t do a lighterpack, but everything I had was in an 18L running vest. Basically just a sleeping bag, pad, shelter, food, and rain jacket. It was probably close to SUL, but I knew the weather was going to likely turn wicked and I was going to be in the middle of nowhere; I didn’t want to go stupid light.
Gear Experiments: There were two pieces of gear I wished to test on this adventure. The first was a Sunday Afternoons hat, as I figured the ridge walking in the sun wouldn’t be enjoyable with just a trucker hat. This was the right call. It worked great. The next test was a pair of white running tights. I’m trying better to use clothing as sun protection, and the tights worked well. I quickly started to wear just the tights, and they breathed fairly well, and had no chafing. They also proved decent protection from bushes. In fact, the only cuts I got where between my socks and the tights; my shins got quite torn up. No sunburns. They weren’t white when I finished.
Gear That Didn’t Work Well: I had several gear malfunctions. The first was my Altra Lone Peak shoes, as I started to get a pretty big blister on my right foot. I thought this was because the shoe was a little too loose, so I tightened it right up. Then I had the skin rub off on the top of my foot. A little leukotape and that problem was solved, but the blister persisted. I rarely got blisters in my Lone Peaks, but had been getting them in the same place for some reason recently, so I pre-taped my foot before heading out. Yes, that’s right, I was getting a blister under tape. Suddenly I realised what was causing the blister: the insole was sliding backwards, which was then putting pressure on my heel. I simply removed the insole, and no more rubbing! Then my right shoe developed a massive hole, which is maybe to be expected, as they have close to (at least) 700km. Finally, I developed life-ending holes in both my Drymax and Injinji socks; the heel of one, the toes of the other. (And I keep my toenails extremely filed down, as per “Fixing Your Feet”).
What would I do differently next time? I would like to avoid Scotts Main Range, and somehow cut up on a parallel track on the other side of the Kowmung River. And then obviously figure out how to get down the Nattai a bit more easily. Alternatively, if I could get my fitness up, and the weather would allow me to go a little bit lighter, I would enjoy being able to run more of the roads. Because of the forecasted weather I had to carry a little bit too much stuff, and the blister was pretty big, or maybe these are the excuses I was telling myself.
Please don’t hesitate reaching out if you wish to do this track and have any questions. This track could be really fun with a group of people without any hard deadlines. This trip report was also cross-posted on r/UltralightAus here: https://www.reddit.com/r/UltralightAus/comments/kox0u9/trip_report_katoomba_to_mittagong_131km/